Method for predicting time sequence of multiple measuring points on gas turbine based on time-varying feature composition
A gas turbine and sequence prediction technology, applied in neural learning methods, neural architectures, computer components, etc., can solve problems such as the strength of correlation, changes in interactive behavior patterns, and failure to take into account, achieving good prediction results and global optimization , Good generalization performance
- Summary
- Abstract
- Description
- Claims
- Application Information
AI Technical Summary
Problems solved by technology
Method used
Image
Examples
Embodiment Construction
[0020] When the gas turbine is running, since each component will encounter different operating states, turbine wear and power changes during operation, the measurement point data of the components will have obvious fluctuations in time series. Since the fluctuation of each component will affect other components inside the gas turbine, the present invention is used to dynamically extract time-varying features in the sequence, and dynamically construct a time-varying association network to improve the time-series prediction model for monitoring the trend of gas turbine operating status.
[0021] The present invention will be further described below in conjunction with the accompanying drawings and specific embodiments, but the following embodiments in no way limit the present invention.
[0022] The design concept of a time-series prediction method for multiple measuring points on a gas turbine based on time-varying feature composition proposed by the present invention is to ext...
PUM
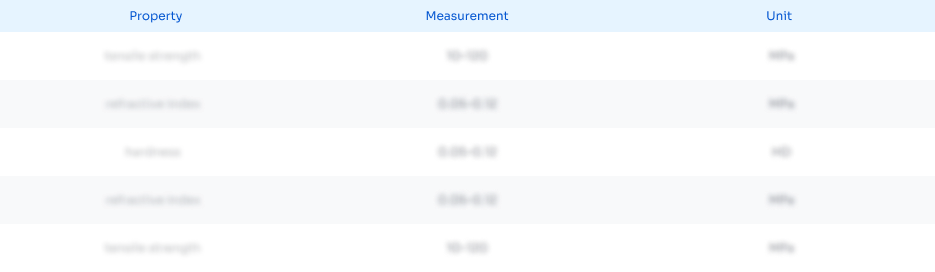
Abstract
Description
Claims
Application Information
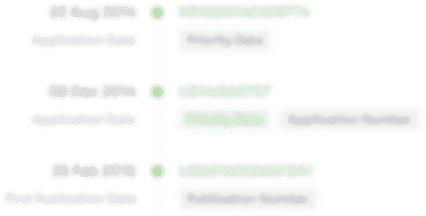
- R&D
- Intellectual Property
- Life Sciences
- Materials
- Tech Scout
- Unparalleled Data Quality
- Higher Quality Content
- 60% Fewer Hallucinations
Browse by: Latest US Patents, China's latest patents, Technical Efficacy Thesaurus, Application Domain, Technology Topic, Popular Technical Reports.
© 2025 PatSnap. All rights reserved.Legal|Privacy policy|Modern Slavery Act Transparency Statement|Sitemap|About US| Contact US: help@patsnap.com