Combined commodity retrieval method and system based on multi-modal pre-training model
A pre-training, multi-modal technology, applied in biological neural network models, business, character and pattern recognition, etc., can solve the problems of low accuracy and achieve the goal of improving accuracy, improving feature representation effect, and strong generalization Effect
- Summary
- Abstract
- Description
- Claims
- Application Information
AI Technical Summary
Problems solved by technology
Method used
Image
Examples
Embodiment 1
[0047] Such as figure 1 As shown, a combined commodity retrieval method based on a multimodal pre-training model, the method includes the following steps:
[0048] S1: Divide the product image into a single product image and a composite product image, wherein the single product image represents only one product, and the composite product image represents multiple independent products;
[0049] S2: Train a combined product image detector to detect each independent product in the combined product image;
[0050] S3: Obtain and combine the feature encoding, position encoding and segmentation encoding of the text mode and picture module in the combined commodity image, thereby learning the embedded representation;
[0051] S4: Construct a multi-modal pre-training model, and use the learned embedding representation as the input of the multi-modal pre-training model;
[0052] S5: The bounding box and bounding box features extracted by the product detector are used as image feature...
Embodiment 2
[0113] Based on the combined product retrieval method of the multi-modal pre-training model described in Embodiment 1, this embodiment also provides a combined product retrieval system of the multi-modal pre-training model. The system includes a sample construction module, an image detection device training module, learning embedding representation module, multimodal pre-training model module, single product feature extraction module, and combined product feature extraction module; among them,
[0114] The sample construction module is used to divide the commodity image into a single product image and a composite product image;
[0115] The image detector training module is used to train an image detector for detecting each independent commodity in the combined commodity image;
[0116] The learning embedding representation module is used to obtain and combine the feature encoding, position encoding and segment encoding of the text mode and picture module in the combined commo...
Embodiment 3
[0125] A computer system includes a memory, a processor, and a computer program stored in the memory and operable on the processor. When the processor executes the computer program, the steps of the method are as follows:
[0126] S1: Divide the product image into a single product image and a composite product image, wherein the single product image represents only one product, and the composite product image represents multiple independent products;
[0127] S2: Train a combined product image detector to detect each independent product in the combined product image;
[0128] S3: Obtain and combine the feature encoding, position encoding and segmentation encoding of the text mode and picture module in the combined commodity image, thereby learning the embedded representation;
[0129] S4: Construct a multi-modal pre-training model, and use the learned embedding representation as the input of the multi-modal pre-training model;
[0130] S5: The bounding box and bounding box fe...
PUM
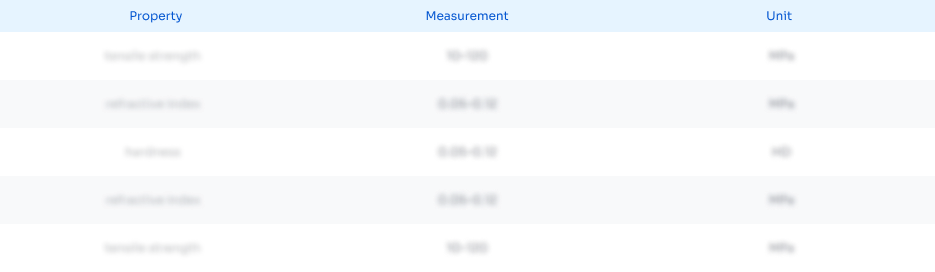
Abstract
Description
Claims
Application Information
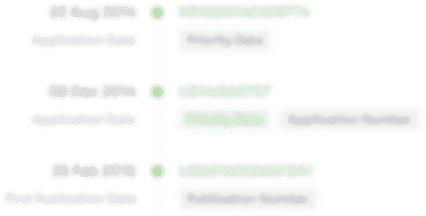
- R&D
- Intellectual Property
- Life Sciences
- Materials
- Tech Scout
- Unparalleled Data Quality
- Higher Quality Content
- 60% Fewer Hallucinations
Browse by: Latest US Patents, China's latest patents, Technical Efficacy Thesaurus, Application Domain, Technology Topic, Popular Technical Reports.
© 2025 PatSnap. All rights reserved.Legal|Privacy policy|Modern Slavery Act Transparency Statement|Sitemap|About US| Contact US: help@patsnap.com