Biological image denoising method based on double-enhanced residual network
A network and image technology, applied in the field of image denoising, can solve problems such as image degradation, training model degradation, and affecting accuracy, and achieve the effect of improving recovery performance and strong application value
- Summary
- Abstract
- Description
- Claims
- Application Information
AI Technical Summary
Problems solved by technology
Method used
Image
Examples
Embodiment Construction
[0027] A biological image denoising method based on a dual enhanced residual network of the present invention is to input the image to be denoised into a denoising model to complete image denoising, and the denoising model is established according to the following steps in turn:
[0028] Step 1: Make a training set
[0029] Step 1.1: Import the BSD500 dataset, add Gaussian noise with known intensity to construct image pairs, select 432 pairs of images, and record them as image sets
[0030] Step 1.2: To image set Carry out the block operation to obtain 4N image blocks. The size of the image after block is 256*256 pixels, which is recorded as the training set
[0031] Step 2: Input the training set image data into the double enhanced residual network to obtain the denoising model
[0032] The double enhanced residual network is as figure 1 As shown, there are two first subnetworks S with the same structure 1 and the second subnetwork S2 , the first subnetwork S 1 Thro...
PUM
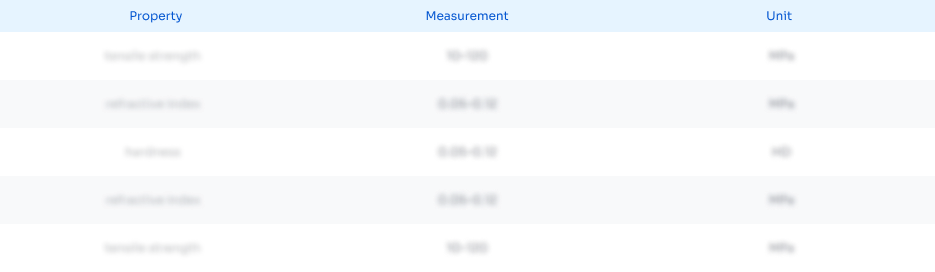
Abstract
Description
Claims
Application Information
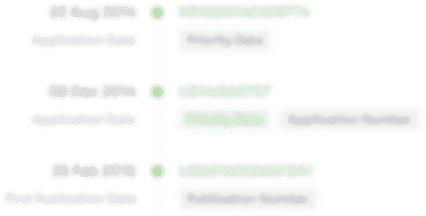
- R&D
- Intellectual Property
- Life Sciences
- Materials
- Tech Scout
- Unparalleled Data Quality
- Higher Quality Content
- 60% Fewer Hallucinations
Browse by: Latest US Patents, China's latest patents, Technical Efficacy Thesaurus, Application Domain, Technology Topic, Popular Technical Reports.
© 2025 PatSnap. All rights reserved.Legal|Privacy policy|Modern Slavery Act Transparency Statement|Sitemap|About US| Contact US: help@patsnap.com