Human body behavior recognition method based on activity graph weighting in multi-cross-domain scene
An activity graph and scene technology, applied in the field of biomedical signal processing, can solve the problems of increasing computational complexity, increasing the difficulty of knowledge transfer, and difficult to apply human behavior recognition, etc., to achieve the effect of low computational complexity
- Summary
- Abstract
- Description
- Claims
- Application Information
AI Technical Summary
Problems solved by technology
Method used
Image
Examples
Embodiment Construction
[0034] In order to make the purpose, technical solution and advantages of the present invention clearer, the present invention will be further described in detail below in conjunction with the implementation methods and accompanying drawings.
[0035] The embodiment of the present invention provides a human behavior recognition method based on activity graph weighting in multiple cross-domain scenarios, including six parts: human physiological signal preprocessing, signal activity graph coding, model pre-training, sample weighting, training model and motion state recognition . The preprocessing of the human physiological signal is as follows: the original human acceleration signal is divided into windows, the bandpass filter is applied to remove the noise, and all samples are standardized; the signal activity map encoding is: the activity map data in different fields have similar distribution characteristics and the activity map data As the input of the model, each dimension o...
PUM
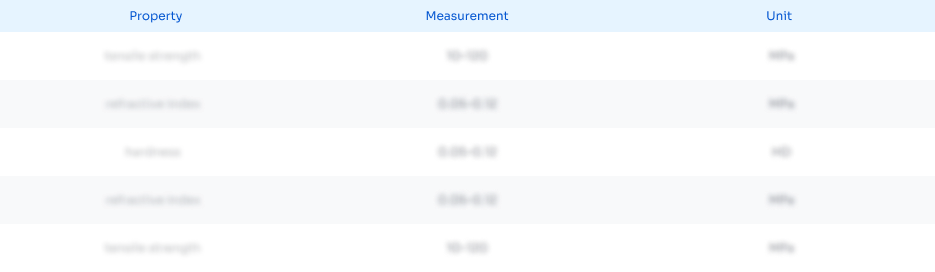
Abstract
Description
Claims
Application Information
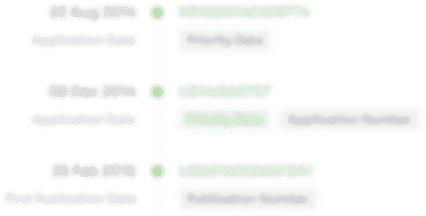
- R&D Engineer
- R&D Manager
- IP Professional
- Industry Leading Data Capabilities
- Powerful AI technology
- Patent DNA Extraction
Browse by: Latest US Patents, China's latest patents, Technical Efficacy Thesaurus, Application Domain, Technology Topic, Popular Technical Reports.
© 2024 PatSnap. All rights reserved.Legal|Privacy policy|Modern Slavery Act Transparency Statement|Sitemap|About US| Contact US: help@patsnap.com