Network traffic scheduling method and system based on deep reinforcement learning
A technology of reinforcement learning and network traffic, applied in the network field, can solve problems such as link congestion, low traversal efficiency, waste of storage space, etc., and achieve a logical and clear effect
- Summary
- Abstract
- Description
- Claims
- Application Information
AI Technical Summary
Problems solved by technology
Method used
Image
Examples
Embodiment Construction
[0037] The scheme of the present invention will be further described below in conjunction with the accompanying drawings and specific embodiments.
[0038] like figure 1 As shown, a network traffic scheduling method based on deep reinforcement learning provided by an embodiment of the present invention includes the following steps:
[0039] Step 1. Network information collection. The topology discovery module in the SDN controller obtains real-time network information for a period of time through the southbound protocol open flow, including port information, bandwidth information, and delay information. The controller collects these information for backup and accumulates information for subsequent information processing.
[0040] Step 2: The data processing module of the management layer processes the collected network information, and obtains status indicators of all links through calculation.
[0041] 1. Bandwidth index: For any link k∈M (M is the link set in the real-ti...
PUM
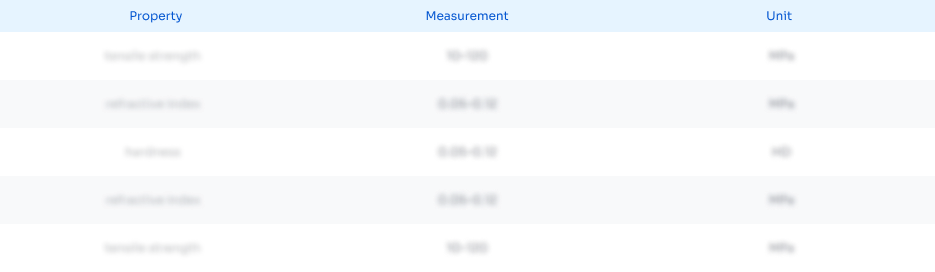
Abstract
Description
Claims
Application Information
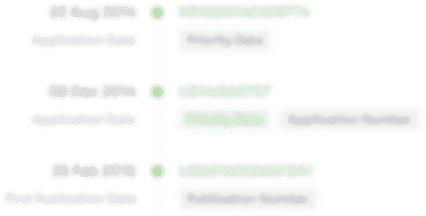
- R&D Engineer
- R&D Manager
- IP Professional
- Industry Leading Data Capabilities
- Powerful AI technology
- Patent DNA Extraction
Browse by: Latest US Patents, China's latest patents, Technical Efficacy Thesaurus, Application Domain, Technology Topic.
© 2024 PatSnap. All rights reserved.Legal|Privacy policy|Modern Slavery Act Transparency Statement|Sitemap