Semi-supervision-based central product quantitative retrieval method
A semi-supervised, retrieval algorithm technology, applied in digital data information retrieval, instrumentation, climate sustainability, etc., can solve the problem of not considering the underlying data structure of unlabeled data, and achieve the effect of improving accuracy and strong robustness
- Summary
- Abstract
- Description
- Claims
- Application Information
AI Technical Summary
Problems solved by technology
Method used
Image
Examples
Embodiment Construction
[0029] The invention proposes a semi-supervised center product quantization image retrieval method. After feature extraction, the space is divided into several subspaces, and then the feature vector is normalized, and the cosine distance is calculated so that the subvector can find the corresponding subspace. The closest codeword in space. In the calculation process, the semi-supervised loss module is used to reduce the quantization error, minimize the empirical error of the labeled data and the embedded error of the unlabeled data, and finally replace the sub-vector quantization with the code word and store it in the product quantization look-up table. The distance calculation is used for image retrieval; the method proposed in the present invention has stronger robustness and improves the accuracy of image retrieval.
[0030] The embodiment of the present invention first establishes a semi-supervised scene through the data set, and the initial goal is to learn a mapping func...
PUM
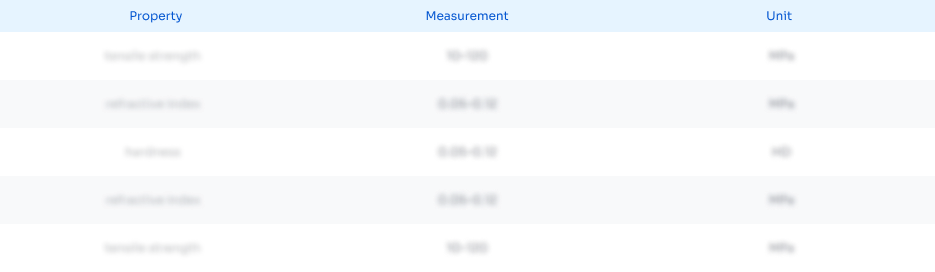
Abstract
Description
Claims
Application Information
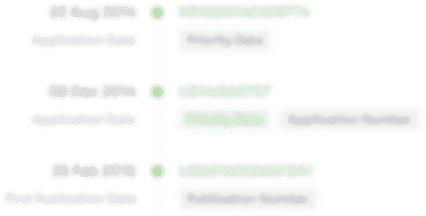
- R&D Engineer
- R&D Manager
- IP Professional
- Industry Leading Data Capabilities
- Powerful AI technology
- Patent DNA Extraction
Browse by: Latest US Patents, China's latest patents, Technical Efficacy Thesaurus, Application Domain, Technology Topic, Popular Technical Reports.
© 2024 PatSnap. All rights reserved.Legal|Privacy policy|Modern Slavery Act Transparency Statement|Sitemap|About US| Contact US: help@patsnap.com