Underwater image enhancement method based on multi-scale dense generative adversarial network and training method of network model
A technology of network model and training method, applied in biological neural network model, image enhancement, image data processing, etc., can solve problems such as difficulty in implementation, poor generalization, and poor implementation effect, so as to ensure diversity and good Restoring, increasing clarity effects
- Summary
- Abstract
- Description
- Claims
- Application Information
AI Technical Summary
Problems solved by technology
Method used
Image
Examples
Embodiment 1
[0059] This embodiment provides a training method for a multi-scale dense generative adversarial network model (hereinafter referred to as the UWGAN-VGG model), and the trained UWGAN-VGG model can effectively enhance underwater images. The steps of UWGAN-VGG model training are as follows.
[0060] 1. Prepare the training data set
[0061] The training data set used in this embodiment is mainly generated by CycleGAN, which is essentially two mirror-symmetric GAN networks, and the cycle-generating adversarial network CycleGAN is an unsupervised network model that does not require paired data sets. The training data set includes the original image in the undistorted air and the distorted image mapped with the original image. These two sets of images do not need to have any correlation, that is, two sets of asymmetric data, and the training data set is asymmetric data. Set; the original undistorted images come from a subset of the ImageNet image set, and the distorted underwater ...
Embodiment 2
[0101] This embodiment provides an underwater image enhancement method based on a multi-scale dense generative adversarial network, and the multi-scale dense generative adversarial network adopts the UWGAN-VGG model trained in Embodiment 1. The enhancement method of the underwater image is as follows:
[0102] Get the original underwater picture;
[0103] The underwater original image is input into the trained multi-scale dense generative adversarial network model for image enhancement processing, and the underwater enhanced image is output.
PUM
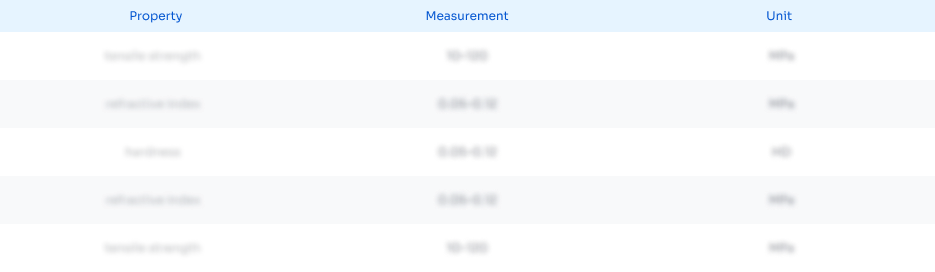
Abstract
Description
Claims
Application Information
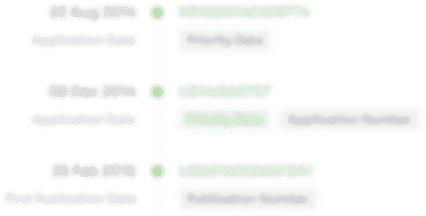
- R&D Engineer
- R&D Manager
- IP Professional
- Industry Leading Data Capabilities
- Powerful AI technology
- Patent DNA Extraction
Browse by: Latest US Patents, China's latest patents, Technical Efficacy Thesaurus, Application Domain, Technology Topic, Popular Technical Reports.
© 2024 PatSnap. All rights reserved.Legal|Privacy policy|Modern Slavery Act Transparency Statement|Sitemap|About US| Contact US: help@patsnap.com