Multi-objective reinforcement learning method and device based on Pareto optimization
A reinforcement learning and multi-objective technology, applied in the direction of specific mathematical models, machine learning, instruments, etc., to achieve fast convergence, good stability, and good performance
- Summary
- Abstract
- Description
- Claims
- Application Information
AI Technical Summary
Problems solved by technology
Method used
Image
Examples
Embodiment Construction
[0051] It should be understood that the specific embodiments described herein are only used to explain the present invention, but not to limit the present invention.
[0052] refer to figure 1 , figure 1 This is a schematic structural diagram of a multi-objective reinforcement learning device based on Pareto optimization of the hardware operating environment involved in the solution of the embodiment of the present invention.
[0053] like figure 1 As shown, the multi-objective reinforcement learning device based on Pareto optimization may include: a processor 1001, such as a central processing unit (Central Processing Unit, CPU), a communication bus 1002, a user interface 1003, a network interface 1004, and a memory 1005. Among them, the communication bus 1002 is used to realize the connection and communication between these components. The user interface 1003 may include a display screen (Display), an input unit such as a keyboard (Keyboard), and the optional user interfa...
PUM
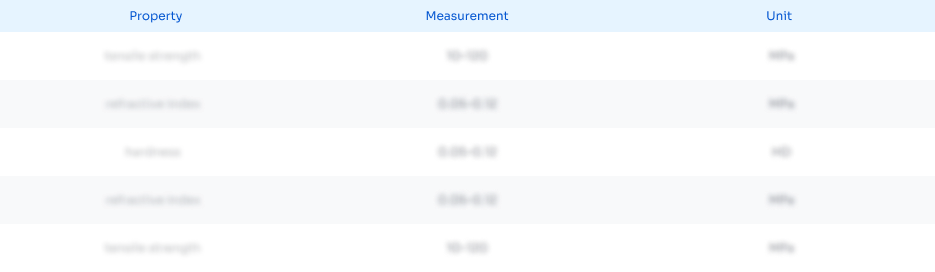
Abstract
Description
Claims
Application Information
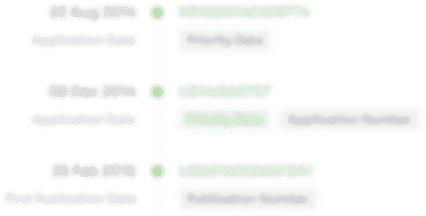
- R&D Engineer
- R&D Manager
- IP Professional
- Industry Leading Data Capabilities
- Powerful AI technology
- Patent DNA Extraction
Browse by: Latest US Patents, China's latest patents, Technical Efficacy Thesaurus, Application Domain, Technology Topic, Popular Technical Reports.
© 2024 PatSnap. All rights reserved.Legal|Privacy policy|Modern Slavery Act Transparency Statement|Sitemap|About US| Contact US: help@patsnap.com