NLP knowledge graph construction method combining information amount and BERT-BiLSTM-CRF
A knowledge map and construction method technology, applied in unstructured text data retrieval, semantic tool creation, machine learning, etc., can solve the problems of insufficient missing value processing, poor classification effect, and insufficient use of data information, etc., to achieve powerful Ability to extract contextual information from text, improve performance
- Summary
- Abstract
- Description
- Claims
- Application Information
AI Technical Summary
Problems solved by technology
Method used
Image
Examples
Embodiment Construction
[0073] The technical solutions in the embodiments of the present application will be clearly and completely described below with reference to the accompanying drawings.
[0074] The implementation process of the technical solution of the present invention includes the following five steps: defining the NLP model layer, acquiring NLP paper data, new word discovery, text multi-classification, and completing the construction of the knowledge map. The overall structure is as follows image 3 shown:
[0075] Step 1), define the NLP knowledge graph schema layer
[0076] The schema layer describes the entities, relationships and attributes in the graph and is the framework of the knowledge graph. In the domain knowledge graph, it is usually necessary to deeply understand the domain knowledge and define the schema layer in combination with the domain data schema. The present invention defines the schema layer of the NLP knowledge graph through a seven-step method.
[0077] (1) Fir...
PUM
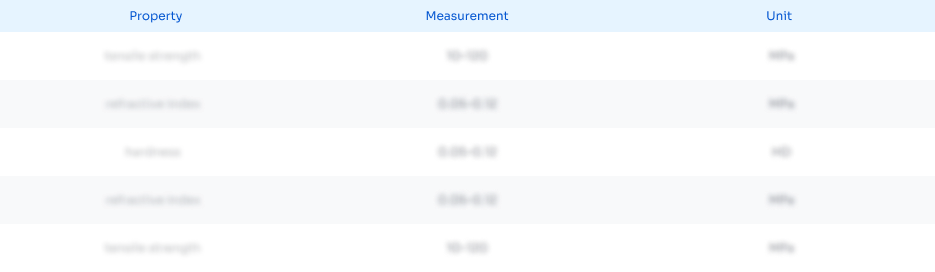
Abstract
Description
Claims
Application Information
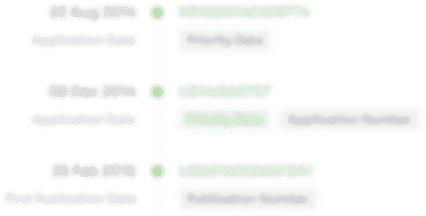
- R&D Engineer
- R&D Manager
- IP Professional
- Industry Leading Data Capabilities
- Powerful AI technology
- Patent DNA Extraction
Browse by: Latest US Patents, China's latest patents, Technical Efficacy Thesaurus, Application Domain, Technology Topic, Popular Technical Reports.
© 2024 PatSnap. All rights reserved.Legal|Privacy policy|Modern Slavery Act Transparency Statement|Sitemap|About US| Contact US: help@patsnap.com