Differential privacy federal modeling method and device for resisting inference attack of semi-honest server
An inferred attack and differential privacy technology, which is applied in the field of differential privacy federated modeling, can solve the problems of inability to resist inferred attacks and low precision of differential privacy models, and achieve the effect of improving the ability to infer attacks, improve accuracy, and improve security
- Summary
- Abstract
- Description
- Claims
- Application Information
AI Technical Summary
Problems solved by technology
Method used
Image
Examples
Embodiment 1
[0049] Embodiments of the present invention provide a differential privacy federation modeling method for resisting semi-honest server inference attacks, including:
[0050] S1: Initialize the global model;
[0051] S2: The semi-honest central server selects a number of data participants to participate in this round of federated training according to a preset probability;
[0052] The data participant selected in S3 downloads the global model, and flexibly fuses the local model trained in the previous round with the global model to obtain the elastically fused local model;
[0053] S4: The selected data participants use local data to perform local private training on the elastically fused local model, obtain the local model after noise disturbance, and then send it to the semi-honest central server;
[0054] S5: The semi-honest central server aggregates the local models perturbed by noise to obtain a perturbed global model.
[0055] Specifically, steps S2 to S5 are performed...
Embodiment 2
[0107] Based on the same inventive concept as the first embodiment, this embodiment provides a differential privacy federation modeling apparatus for resisting semi-honest server inference attacks, including:
[0108] The initialization module is used to initialize the global model;
[0109] The data participant selection module is used to select a number of data participants to participate in this round of federated training according to a preset probability;
[0110] The elastic fusion module is used to download the global model, and flexibly fuse the local model trained in the previous round with the global model to obtain the elastically fused local model;
[0111] The local secret training module is used to perform local private training on the elastically fused local model by using local data, obtain the local model after noise disturbance, and then send it to the semi-honest central server;
[0112] The aggregation module is used to aggregate the local models perturbed...
PUM
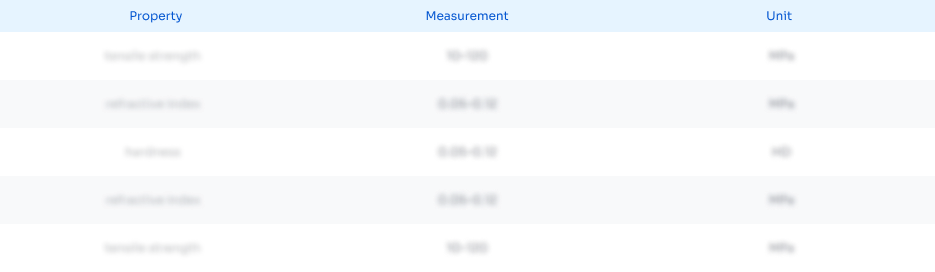
Abstract
Description
Claims
Application Information
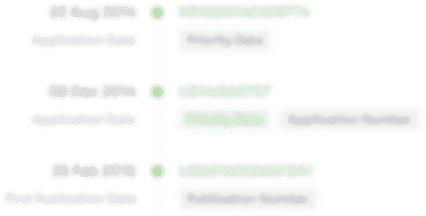
- R&D
- Intellectual Property
- Life Sciences
- Materials
- Tech Scout
- Unparalleled Data Quality
- Higher Quality Content
- 60% Fewer Hallucinations
Browse by: Latest US Patents, China's latest patents, Technical Efficacy Thesaurus, Application Domain, Technology Topic, Popular Technical Reports.
© 2025 PatSnap. All rights reserved.Legal|Privacy policy|Modern Slavery Act Transparency Statement|Sitemap|About US| Contact US: help@patsnap.com