SENet-based gastric cancer pathological section image segmentation prediction method
A technology of pathological sectioning and image segmentation, which is applied in the field of image processing, can solve the problems of high complexity and poor detection effect of small lesions, achieve the effect of increasing the depth of representation, improving the accuracy of detection, and solving the effects of computing resources
- Summary
- Abstract
- Description
- Claims
- Application Information
AI Technical Summary
Problems solved by technology
Method used
Image
Examples
Embodiment 1
[0064] like figure 1 As shown, this embodiment provides a method for segmenting and predicting gastric cancer pathological slice images based on SENet, including the following steps:
[0065] S1: Extract the foreground tissue area based on the Otsu algorithm and use the foreground mask map and slice-level real labels to filter out cavities and backgrounds, and generate image blocks and corresponding image block-level real labels based on the dense tiling algorithm. The specific steps include:
[0066] According to the annotation file, the positive area annotation mask map is generated, the foreground tissue is extracted from the pathological slice based on the Otsu algorithm to generate the tissue mask map, and then the annotation mask map and the tissue mask map are ANDed to obtain the annotation mask for filtering out the cavity picture;
[0067] Set a square sliding window in the upper left corner of the original slice and labeling mask image, and start sliding with the wi...
Embodiment 2
[0094] This embodiment provides an SENet-based gastric cancer pathological slice image segmentation prediction system, including: an image preprocessing module, a multi-channel convolution unit construction unit, a network model construction module, a training module, and a prediction module;
[0095] In this embodiment, the image preprocessing module is used to extract the foreground tissue region based on the Otsu algorithm, filter out the cavity and the background by using the foreground mask map and the slice-level real label to do an AND operation, and generate image blocks and corresponding images based on the dense tiling algorithm. block-level ground truth labels;
[0096] In this embodiment, the multi-channel convolution unit construction unit is used to construct a multi-channel convolution unit based on the SENet module using depthwise separable convolution, standard convolution, concatenation operation and sum operation;
[0097] In this embodiment, the network mod...
Embodiment 3
[0101] This embodiment provides a storage medium. The storage medium may be a storage medium such as a ROM, a RAM, a magnetic disk, an optical disc, etc., and the storage medium stores one or more programs. A segmentation prediction method for gastric cancer pathological slice images.
PUM
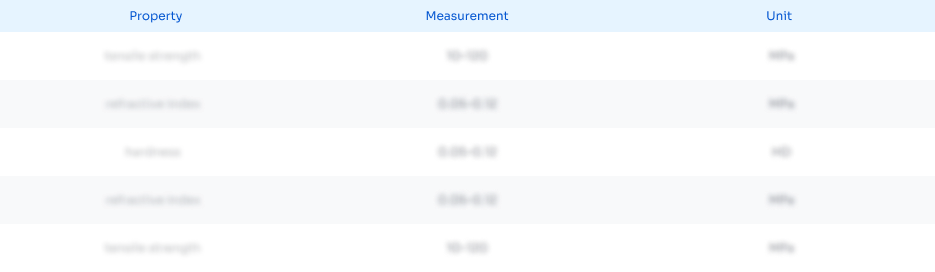
Abstract
Description
Claims
Application Information
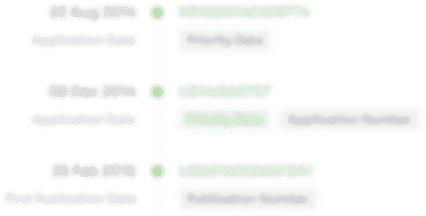
- Generate Ideas
- Intellectual Property
- Life Sciences
- Materials
- Tech Scout
- Unparalleled Data Quality
- Higher Quality Content
- 60% Fewer Hallucinations
Browse by: Latest US Patents, China's latest patents, Technical Efficacy Thesaurus, Application Domain, Technology Topic, Popular Technical Reports.
© 2025 PatSnap. All rights reserved.Legal|Privacy policy|Modern Slavery Act Transparency Statement|Sitemap|About US| Contact US: help@patsnap.com