Multivariable time sequence anomaly detection method and system based on graph neural network
A time series, anomaly detection technology, applied in neural learning methods, biological neural network models, neural architectures, etc., can solve problems such as large changes in outliers, and achieve the effects of reducing fluctuations, improving performance, and improving accuracy
- Summary
- Abstract
- Description
- Claims
- Application Information
AI Technical Summary
Problems solved by technology
Method used
Image
Examples
Embodiment Construction
[0048] In order to make the above-mentioned features and effects of the present invention more clearly and comprehensible, embodiments are given below, and detailed descriptions are given below in conjunction with the accompanying drawings.
[0049] First, a time series model is constructed based on the theory of probabilistic graph models to model multi-dimensional time series relationships, so as to realize a dynamic graph neural network; The mechanism realizes the aggregation of graph neural network node features; at the same time, the normalized time alignment measure (TAM) is used to approximate the adjacency matrix, and the reconstruction error of the adjacency matrix is explicitly introduced into the loss function, so that the graph embedding represents the division of the adjacency matrix. In addition to the domain structure, there is also the similarity of the node data itself. Then, according to the difference in the distribution of each node and its neighbors, the...
PUM
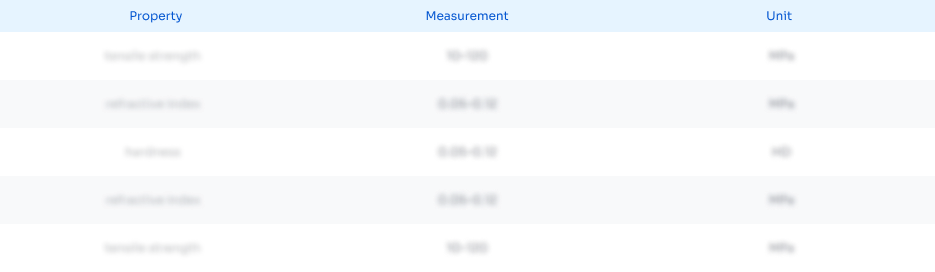
Abstract
Description
Claims
Application Information
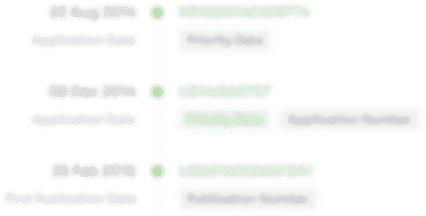
- R&D Engineer
- R&D Manager
- IP Professional
- Industry Leading Data Capabilities
- Powerful AI technology
- Patent DNA Extraction
Browse by: Latest US Patents, China's latest patents, Technical Efficacy Thesaurus, Application Domain, Technology Topic.
© 2024 PatSnap. All rights reserved.Legal|Privacy policy|Modern Slavery Act Transparency Statement|Sitemap