Small sample target detection method for structured filter learning
A target detection and filter technology, applied in the field of small-sample target detection, can solve the problems of missing quantity, sufficient data, time-consuming and labor-intensive problems, and achieve the effect of improving feature expression ability and performance enhancement
- Summary
- Abstract
- Description
- Claims
- Application Information
AI Technical Summary
Problems solved by technology
Method used
Image
Examples
Embodiment Construction
[0018] like figure 1 As shown, a small sample target detection method for structured filter learning of the present invention includes the following steps:
[0019] Step 1, prepare a small sample target detection data set and a base class target detection data set;
[0020] Step 2, build a network structure. The backbone feature extraction network uses CSPDarknet53 and introduces a general feature enhancement module; uses the structured filter S_conv to construct the convolution unit S_CBL and the Yolo detection head S_Yolo; and then combines the feature fusion network structure of YOLOv4 to build a small sample target detection for structured filter learning deep neural network;
[0021] Step 3, the first stage of training, use the base class data set to train the network;
[0022] Step 4, the second stage of training, the backbone feature extraction network CSPDarknet53 and the general feature enhancement module use the weights pre-trained in the base class target detecti...
PUM
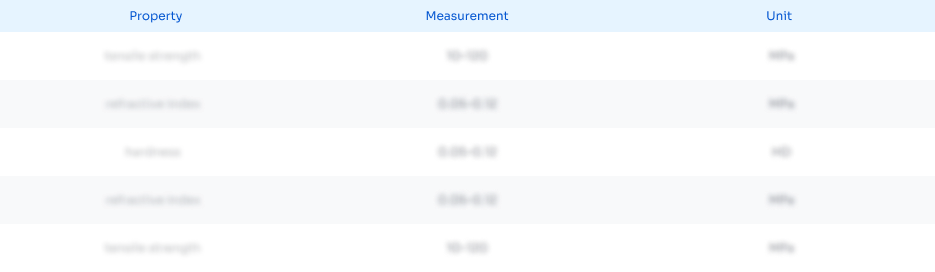
Abstract
Description
Claims
Application Information
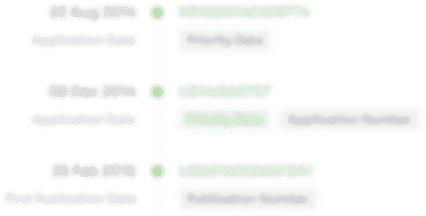
- R&D Engineer
- R&D Manager
- IP Professional
- Industry Leading Data Capabilities
- Powerful AI technology
- Patent DNA Extraction
Browse by: Latest US Patents, China's latest patents, Technical Efficacy Thesaurus, Application Domain, Technology Topic, Popular Technical Reports.
© 2024 PatSnap. All rights reserved.Legal|Privacy policy|Modern Slavery Act Transparency Statement|Sitemap|About US| Contact US: help@patsnap.com