Detection device and method for multi-class targets
A detection device and detection method technology, which can be applied to instruments, character and pattern recognition, computer parts and other directions, and can solve problems such as difficulty in classifier training.
- Summary
- Abstract
- Description
- Claims
- Application Information
AI Technical Summary
Problems solved by technology
Method used
Image
Examples
no. 2 example
[0057] According to the second embodiment of the present invention, the detection device for detecting multi-category target data is designed as a cascade structure (Cascade) classifier in which a plurality of classifiers are connected in series. To this end, the classifiers at all levels of the cascade classifier (SC k ) is artificially designed as a predetermined multi-layer structure (the first multi-layer structure in the present invention). Set the most detailed categories (for example, r categories, r is a natural number greater than 1) at the bottom, and then merge these categories into a few larger categories at a higher level according to a predetermined similarity standard, Then it is merged step by step up to the highest level, such as a large category.
[0058] Figure 5a and 5b Shows the use of a class tree structure CT to represent sample class changes during training. Figure 5a , a total of 7 types of object samples participate in the training, these 7 type...
no. 3 example
[0080] In the third embodiment, cars, buses and trucks are used as objects to be detected, and a more detailed classification (training) method for cascaded classifiers is described.
[0081] First, prepare three types of positive sample sets (car images) P(C i )(i=1, 2, 3) correspond to cars, buses and trucks respectively, and combine the three types of positive samples into one type of positive sample set P(C 0 ), the structure of the sample category tree is as follows Figure 5b shown; training from P(C i )(i=0), when it is necessary to split the positive sample category, P(C i )(i=0) is split into P(C i )(i=1, 2, 3); and set all kinds of expected training targets: detection rate D i and the total false detection rate F i ;
[0082] Next, prepare the feature pool, and apply the Haar-like feature prototype to a 32×32 (pixel) image to obtain hundreds of thousands of specific features.
[0083] Then train classifiers SC at all levels step by step 1 to SC n . Such as ...
PUM
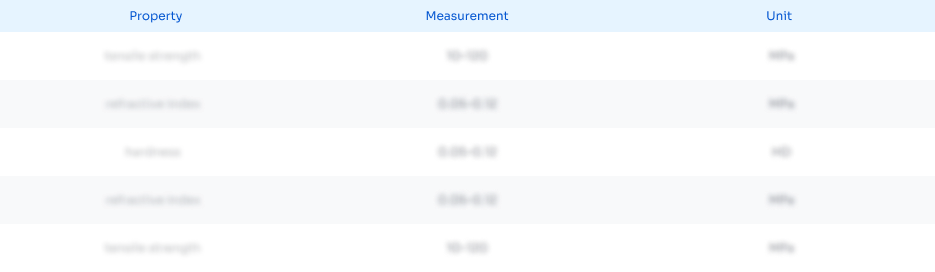
Abstract
Description
Claims
Application Information
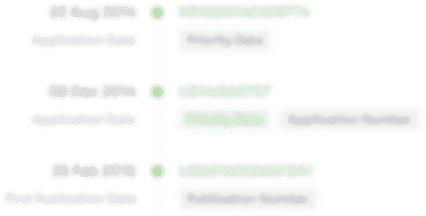
- R&D Engineer
- R&D Manager
- IP Professional
- Industry Leading Data Capabilities
- Powerful AI technology
- Patent DNA Extraction
Browse by: Latest US Patents, China's latest patents, Technical Efficacy Thesaurus, Application Domain, Technology Topic, Popular Technical Reports.
© 2024 PatSnap. All rights reserved.Legal|Privacy policy|Modern Slavery Act Transparency Statement|Sitemap|About US| Contact US: help@patsnap.com