Local spline embedding-based orthogonal semi-monitoring subspace image classification method
A technology of local spline embedding and classification method, applied in computer parts, instruments, character and pattern recognition, etc., can solve problems such as time-consuming
- Summary
- Abstract
- Description
- Claims
- Application Information
AI Technical Summary
Problems solved by technology
Method used
Image
Examples
Embodiment
[0062] 1. Select n sample data for each image data set as a training set, the training set includes training samples with labeled information and training samples without labeled information, and the rest as a test set;
[0063] 2. Use the training samples with labeled information to construct the inter-class scatter matrix and intra-class scatter matrix:
[0064] Given a training set X={x 1 ,..,x l , x l+1 ,...,x n},in i=1,...,n, the first l samples With category labeling information remaining n-l samples Not marked. Use training samples that contain labeled information Construct the between-class scatter matrix S b and the intra-class scatter matrix S w :
[0065] S b = Σ k = 1 c l k ( μ ( k ) - ...
PUM
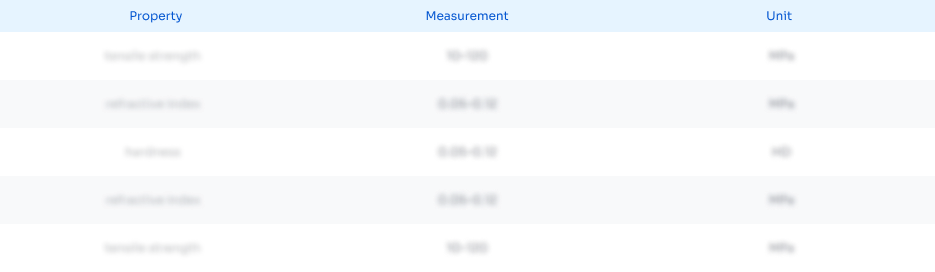
Abstract
Description
Claims
Application Information
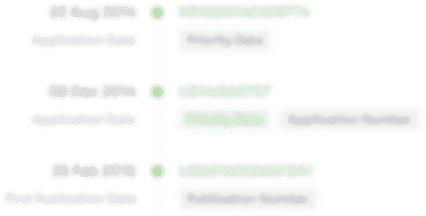
- R&D
- Intellectual Property
- Life Sciences
- Materials
- Tech Scout
- Unparalleled Data Quality
- Higher Quality Content
- 60% Fewer Hallucinations
Browse by: Latest US Patents, China's latest patents, Technical Efficacy Thesaurus, Application Domain, Technology Topic, Popular Technical Reports.
© 2025 PatSnap. All rights reserved.Legal|Privacy policy|Modern Slavery Act Transparency Statement|Sitemap|About US| Contact US: help@patsnap.com