Remote sensing classification method for binary tree multi-category support vector machines
A technology of support vector machine and classification method, which is applied in the field of binary tree multi-class support vector machine remote sensing classification, can solve the problems that the training speed is not as good as the first class method, and the classification accuracy is not superior, so as to achieve fast classification speed, excellent classification accuracy, and improved The effect of classification accuracy
- Summary
- Abstract
- Description
- Claims
- Application Information
AI Technical Summary
Problems solved by technology
Method used
Image
Examples
Embodiment 1
[0019] Embodiment 1: All categories are first divided into two subcategories, and then the subcategories are further divided into two subcategories, and the cycle continues until a single category is obtained, so that a series of SVMs will be finally obtained The resulting binary classification tree completes the construction of the binary tree multi-class support vector machine classifier; in the classification process, the classification error appears in the classifier far away from the root node, and the class with the largest JM distance is first separated; using the established Binary tree multi-class support vector machine classifier to realize remote sensing image classification; improve the accuracy and reliability of remote sensing image classification.
[0020] The specific implementation steps are:
[0021] (1) Using training samples of each category, according to the formula , calculate the separability measure between any two classes, combine the separability me...
Embodiment 2
[0031] Example 2: Using OMIS in Changping District, Beijing Experiment with hyperspectral images.
[0032] OMIS The hyperspectral image size is 512 rows, 512 columns, and 64 bands. Using the preprocessed data, select the training samples for training, and construct such as figure 1 The binary tree multi-class support vector machine model shown has a classification accuracy of 96.52%, a Kappa coefficient of 0.8335, and a classification time of 1.0310S. The accuracy of the present invention is compared with that of existing methods.
[0033] Table 1 Different multi-class support vector machine classification results
[0034]
[0035] It can be seen that among the above various classification methods, the J-M distance-based binary tree SVM classification method proposed by the present invention has the highest precision. Compared with the conventional one-to-residue and one-to-one comparisons, both accuracy and test time are greatly improved.
Embodiment 3
[0036] Example 3: Experiments were conducted using Hyperion hyperspectral images in the California region of the United States.
[0037] The Hyperion experiment data is the hyperspectral image of EO-1 Hyperion in California, USA. Table 2 is the accuracy comparison of different methods.
[0038] Table 2 Different multi-class support vector machine classification results of Hyperion data
[0039]
[0040] It can be seen that when the binary tree multi-class support vector machine method based on JM distance is used for remote sensing image classification, it has the advantages of simple structure, easy training, fast convergence speed, and high classification accuracy. Compared with angle mapping and minimum distance classification methods, it has certain advantages, and it is an effective remote sensing image classification method.
[0041] Others are the same as in Example 1.
PUM
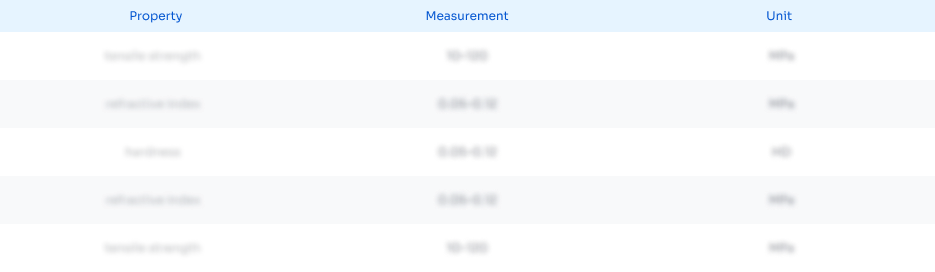
Abstract
Description
Claims
Application Information
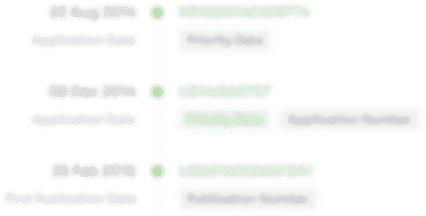
- R&D Engineer
- R&D Manager
- IP Professional
- Industry Leading Data Capabilities
- Powerful AI technology
- Patent DNA Extraction
Browse by: Latest US Patents, China's latest patents, Technical Efficacy Thesaurus, Application Domain, Technology Topic.
© 2024 PatSnap. All rights reserved.Legal|Privacy policy|Modern Slavery Act Transparency Statement|Sitemap