Markov random field model and non-local prior based image registration method
A random field model and image registration technology, which is applied to computer parts, character and pattern recognition, instruments, etc., can solve the problem that the algorithm is stuck in local extremum and the amount of mutual information does not consider the image space and direction information, etc. Achieve the effect of improving registration accuracy, strong robustness, and reducing the possibility of falling into local extremum
- Summary
- Abstract
- Description
- Claims
- Application Information
AI Technical Summary
Problems solved by technology
Method used
Image
Examples
Embodiment Construction
[0024] The specific embodiment of the present invention is as Figure 1 ~ Figure 3 As shown, in this embodiment, a set of CT images scanned before and after contrast agent injection into the abdomen of a human body (see image 3 a~3b), explain the working steps of the present invention in detail.
[0025] In step 1, the CT images scanned before and after contrast agent injection are respectively read in as target images and floating images to be registered. By linear transformation (I-I min )×255 / (I max -I min ) Transform the gray value of all pixels of the target image and the floating image into the range of 0-255, where I is the gray value of the image, that is, when performing linear transformation on the CT image scanned before injecting the contrast agent, I is the injection contrast agent The gray value of the CT image scanned before the injection of the contrast agent; when the linear transformation is performed on the CT image scanned after the injection of the co...
PUM
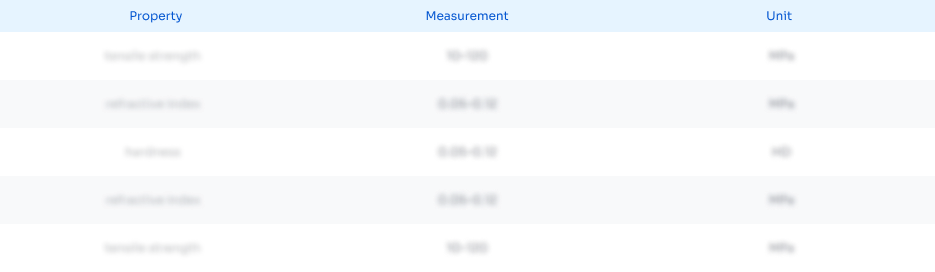
Abstract
Description
Claims
Application Information
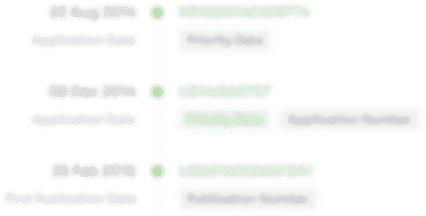
- R&D Engineer
- R&D Manager
- IP Professional
- Industry Leading Data Capabilities
- Powerful AI technology
- Patent DNA Extraction
Browse by: Latest US Patents, China's latest patents, Technical Efficacy Thesaurus, Application Domain, Technology Topic.
© 2024 PatSnap. All rights reserved.Legal|Privacy policy|Modern Slavery Act Transparency Statement|Sitemap