Natural image noise removal method based on dual redundant dictionary learning
A dictionary learning, natural image technology, applied in the field of image processing, can solve the problem of rough error control method, loss of texture details, inability to effectively approximate the edge and detail information of the original image, etc., to achieve the effect of fine error control and noise removal
- Summary
- Abstract
- Description
- Claims
- Application Information
AI Technical Summary
Problems solved by technology
Method used
Image
Examples
Embodiment Construction
[0035] refer to figure 1 , the implementation steps of the present invention are as follows:
[0036] Step 1: Construct a multi-scale redundant stationary wavelet dictionary R.
[0037] First, the Haar wavelet function is selected and translated accordingly to obtain a multi-scale redundant stationary wavelet dictionary R; the image Y to be denoised is expanded under the multi-scale redundant stationary wavelet dictionary R, and the number of decomposition layers is set to r, then the expansion coefficient scale-by-scale Divide into N=3r+1 blocks, and obtain the coefficient β=[β 1 , β 2 ,...,β N ], j=1, 2...N, set the multi-scale coefficient component β on the jth block j in another redundant dictionary D j The following has a sparse representation, which satisfies the following formula: Y=X+n=R*β+n=R*D*A+n, where Y is the image to be denoised, X is the clear image, n is the noise, and β is The sparse representation coefficients of Y under the multi-scale redundant sta...
PUM
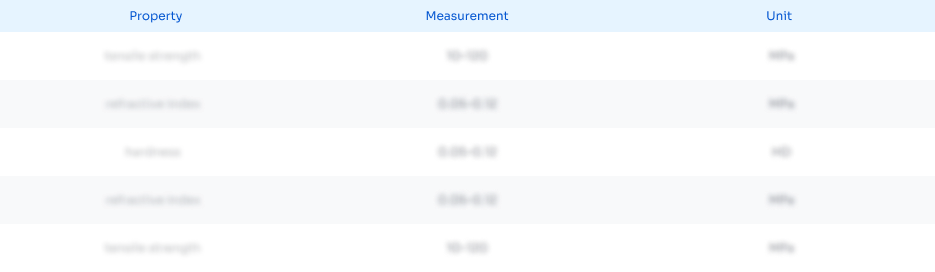
Abstract
Description
Claims
Application Information
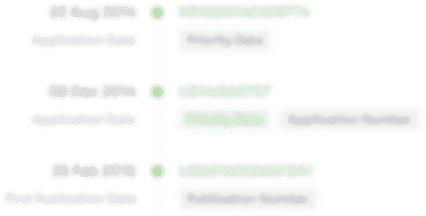
- R&D Engineer
- R&D Manager
- IP Professional
- Industry Leading Data Capabilities
- Powerful AI technology
- Patent DNA Extraction
Browse by: Latest US Patents, China's latest patents, Technical Efficacy Thesaurus, Application Domain, Technology Topic, Popular Technical Reports.
© 2024 PatSnap. All rights reserved.Legal|Privacy policy|Modern Slavery Act Transparency Statement|Sitemap|About US| Contact US: help@patsnap.com