Semi-supervised dimension reduction-based hyper-spectral image classification method
A hyperspectral image and dimensionality reduction technology, applied in the field of image processing, can solve problems such as difficulty, time-consuming and labor-intensive acquisition of category information, difficulty in obtaining classification results, etc.
- Summary
- Abstract
- Description
- Claims
- Application Information
AI Technical Summary
Problems solved by technology
Method used
Image
Examples
Embodiment Construction
[0033] refer to figure 1 , the specific implementation steps of the present invention include:
[0034] Step 1, input the hyperspectral image, select the same amount of pixels from each type of pixels in the hyperspectral image as marked pixels, the total number of marked pixels is N, and the rest of the hyperspectral image m pixels are used as unmarked pixels, and the gray value of each band of each pixel is used as the feature vector of the pixel. Each pixel is a sample, and the original feature dimension of the sample is D.
[0035] Step 2, select the labeled training set X, the test set Y and the total training set S.
[0036] 2a) Use N labeled samples to form a labeled training set Its corresponding category label set is where x i Indicates the i-th labeled training sample of the labeled training set, and each labeled training sample is represented by a column vector, l i is the category label to which the i-th labeled training sample belongs, N is the total number...
PUM
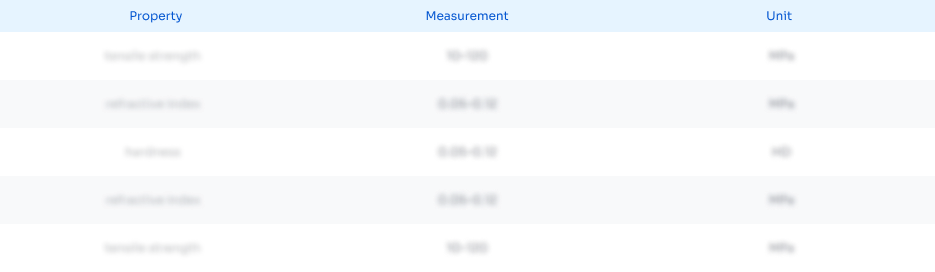
Abstract
Description
Claims
Application Information
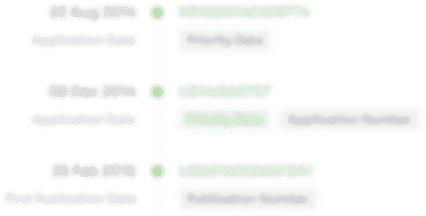
- R&D Engineer
- R&D Manager
- IP Professional
- Industry Leading Data Capabilities
- Powerful AI technology
- Patent DNA Extraction
Browse by: Latest US Patents, China's latest patents, Technical Efficacy Thesaurus, Application Domain, Technology Topic.
© 2024 PatSnap. All rights reserved.Legal|Privacy policy|Modern Slavery Act Transparency Statement|Sitemap