Nonlinear Facial Motion Manifold Learning Method Based on Statistical Shape Theory
A technology of statistical shape and learning method, applied in the field of image processing, can solve the problem of not considering the characteristics of facial movement, and achieve the effect of overcoming inaccurate distance calculation and good approximation effect.
- Summary
- Abstract
- Description
- Claims
- Application Information
AI Technical Summary
Problems solved by technology
Method used
Image
Examples
Embodiment Construction
[0027] The present invention is a nonlinear facial motion manifold learning method based on statistical shape theory; including two parts: preprocessing based on statistical shape theory and facial motion manifold learning using a Gaussian process hidden variable model; wherein:
[0028] 1. Regarding preprocessing based on statistical shape theory, the steps are as follows:
[0029] Assume Ω={ω i |i=1, 2...N} is a facial shape motion sequence, where ω i ={(x i1 ,y i1 ), (x i2 ,y i2 )...(x iM ,y iM )} represents a frame of face shape consisting of M points.
[0030] Step 1: Demeanize the shape of each frame in the facial motion sequence Ω, and first obtain the center position of the facial shape of each frame (x 0 ,y 0 ), Then remove the center position information from the shape data, ie x ij '=x ij -x 0 ,y ij '=yij -y 0 Make Σ j x ij ′ + ...
PUM
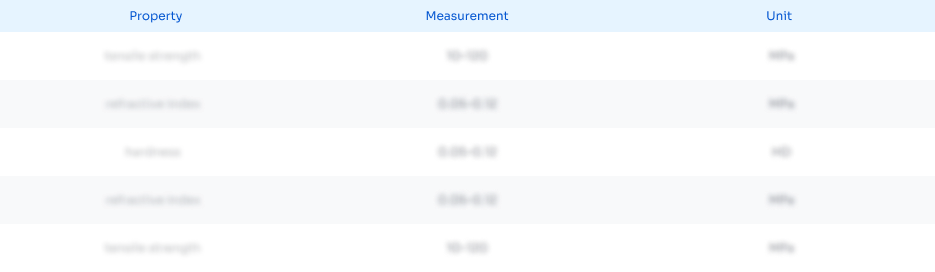
Abstract
Description
Claims
Application Information
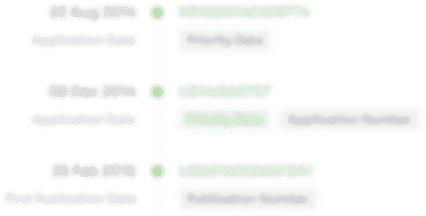
- R&D Engineer
- R&D Manager
- IP Professional
- Industry Leading Data Capabilities
- Powerful AI technology
- Patent DNA Extraction
Browse by: Latest US Patents, China's latest patents, Technical Efficacy Thesaurus, Application Domain, Technology Topic, Popular Technical Reports.
© 2024 PatSnap. All rights reserved.Legal|Privacy policy|Modern Slavery Act Transparency Statement|Sitemap|About US| Contact US: help@patsnap.com