Cluster anomaly detection method of combustion gas turbine
A gas turbine and anomaly detection technology, applied in the direction of measuring devices, instruments, etc., can solve problems such as excessive information volume, limited fault monitoring level, and insufficient early warning technology
- Summary
- Abstract
- Description
- Claims
- Application Information
AI Technical Summary
Problems solved by technology
Method used
Image
Examples
specific Embodiment approach 1
[0019] Specific implementation mode one: as figure 1 As shown, the specific process of the gas turbine cluster anomaly detection method described in this embodiment is:
[0020] Step 1. Obtain monitoring data and input parameters: Obtain G monitoring data at different times from the monitoring software of the gas turbine, and use P below k Indicates the monitoring value at the kth moment, where 1≤k≤G; and input parameters: weight vector The number of iterations LT, the update parameter λ, and the abnormal reference ratio β;
[0021] Step 2, extracting the feature quantities that characterize the characteristics of each different moment: select the feature quantity PF from the monitoring data k (l), where 1≤l≤29, which corresponds to the values of the following 29 measuring points: gearbox vibration, generator DEX vibration, generator DEY vibration, generator EEX vibration, generator EEY vibration, gas generator speed , Generator total actual power, 3# bearing Y vibration...
specific Embodiment approach 2
[0030] Specific implementation mode two: as figure 1 As shown, the clustering anomaly detection method described in this embodiment is characterized in that:
[0031] In step 4, the cluster center sample to which each sample belongs is obtained from the G samples fc k = arg f max { a ( k , f ) + r ( k , f ) } The specific method is:
[0032] Step 41: Obtain the similarity between the bth sample and the gth sample Sim ( b , g ) = - Dist ( PF ...
PUM
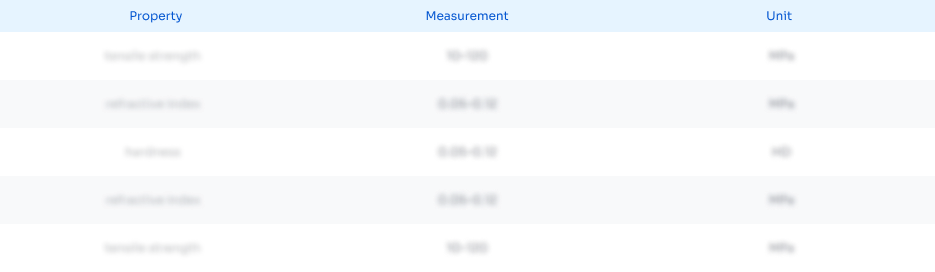
Abstract
Description
Claims
Application Information
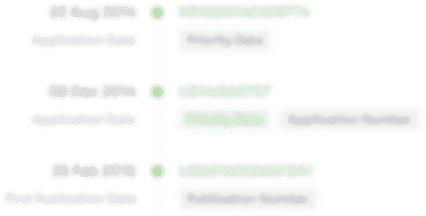
- R&D Engineer
- R&D Manager
- IP Professional
- Industry Leading Data Capabilities
- Powerful AI technology
- Patent DNA Extraction
Browse by: Latest US Patents, China's latest patents, Technical Efficacy Thesaurus, Application Domain, Technology Topic, Popular Technical Reports.
© 2024 PatSnap. All rights reserved.Legal|Privacy policy|Modern Slavery Act Transparency Statement|Sitemap|About US| Contact US: help@patsnap.com