Residual-based ultra-resolution image reconstruction method
A super-resolution reconstruction and low-resolution technology, applied in the field of residual-based image super-resolution reconstruction, can solve problems such as artificial traces and unsatisfactory results
- Summary
- Abstract
- Description
- Claims
- Application Information
AI Technical Summary
Problems solved by technology
Method used
Image
Examples
Embodiment Construction
[0034] The present invention will be described in detail below in conjunction with the accompanying drawings and implementation examples.
[0035] For a color RGB image, first convert it to a YUV image, perform super-resolution reconstruction on the Y component, and the UV component is enlarged by interpolation, and then convert the YUV image into an RGB image; for a grayscale image, directly on the grayscale image Perform super-resolution reconstruction.
[0036] Performs a 3x upscaling on the input low-resolution image. Divide the low-resolution image into several image sub-blocks with a size of 3×3, and the corresponding high-resolution image sub-block size is 9×9. In order to maintain the compatibility between the image sub-blocks, the low-resolution image sub-blocks If a block takes 1 overlapping pixel, the corresponding high-resolution image sub-block overlaps by 3 pixels.
[0037] Step (1) Calculate the residual
[0038] The low-resolution sample image y used for tra...
PUM
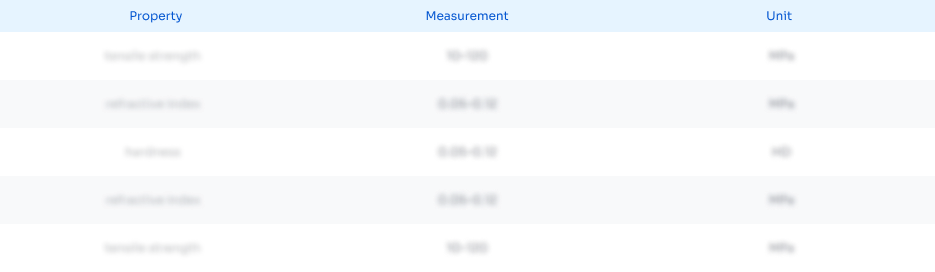
Abstract
Description
Claims
Application Information
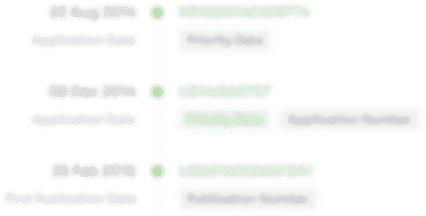
- R&D Engineer
- R&D Manager
- IP Professional
- Industry Leading Data Capabilities
- Powerful AI technology
- Patent DNA Extraction
Browse by: Latest US Patents, China's latest patents, Technical Efficacy Thesaurus, Application Domain, Technology Topic, Popular Technical Reports.
© 2024 PatSnap. All rights reserved.Legal|Privacy policy|Modern Slavery Act Transparency Statement|Sitemap|About US| Contact US: help@patsnap.com