Image super-resolution reconstruction method based on double-dictionary learning
A double-dictionary and super-resolution technology, applied in the field of image processing, can solve the problems of high-resolution images lacking detailed information, long image reconstruction time, blurred image edges, etc.
- Summary
- Abstract
- Description
- Claims
- Application Information
AI Technical Summary
Problems solved by technology
Method used
Image
Examples
Embodiment Construction
[0048] refer to figure 1 , the specific implementation steps of the present invention are as follows:
[0049] Step 1, input the low-resolution image X to be processed L ,Such as Figure 4 As shown, the size of the image is m×n, where m is the number of image rows, n is the number of image columns, and the magnification is set to 2, and the low-resolution image to be processed is X L Carry out block division with a size of 3×3, overlap 2 pixels between adjacent blocks, and obtain G low-resolution image blocks P to be processed l (i), i=1, . . . , G.
[0050] Step 2, input 5 high-resolution training images and 5 corresponding low-resolution training images, among which 5 high-resolution training images are as follows figure 2 As shown, the 5 low-resolution training images are as follows image 3 As shown, use training images to construct 5 high-resolution dictionaries D h1 ,D h2 ,...,D h5 And the corresponding 5 low-resolution dictionaries D l1 ,D l2 ,...,D l5 .
...
PUM
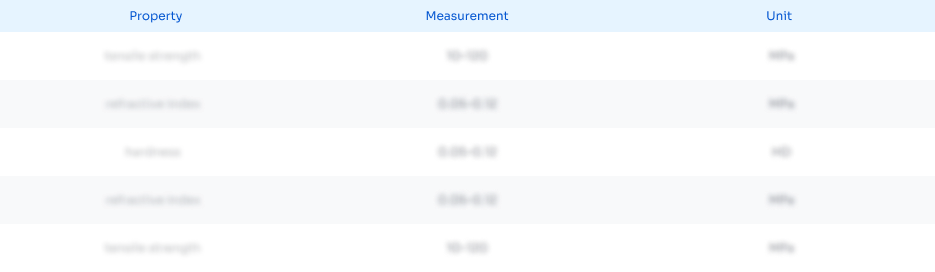
Abstract
Description
Claims
Application Information
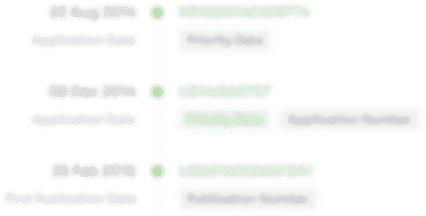
- R&D
- Intellectual Property
- Life Sciences
- Materials
- Tech Scout
- Unparalleled Data Quality
- Higher Quality Content
- 60% Fewer Hallucinations
Browse by: Latest US Patents, China's latest patents, Technical Efficacy Thesaurus, Application Domain, Technology Topic, Popular Technical Reports.
© 2025 PatSnap. All rights reserved.Legal|Privacy policy|Modern Slavery Act Transparency Statement|Sitemap|About US| Contact US: help@patsnap.com