A semi-supervised hyperspectral image dimensionality reduction method based on improved k-means clustering
A K-means and hyperspectral technology, which is applied in the field of hyperspectral remote sensing image processing, can solve the problems of not distinguishing the difference in importance, achieve fast dimensionality reduction, high classification accuracy, and reduce the amount of calculation
- Summary
- Abstract
- Description
- Claims
- Application Information
AI Technical Summary
Problems solved by technology
Method used
Image
Examples
Embodiment 1
[0056] Example 1: Experimental data The images of the Indian Pines area acquired by the AVIRIS sensor in June 1992 in the northwestern part of Indiana. The data covers 220 spectral bands from 0.4 to 2.4um spectral interval, with a spectral resolution of 10nm and a spatial resolution of 20m. After preprocessing the dataset to remove water absorption and low SNR bands, 202 bands remained. The size of the data is 145×145, and it contains 16 different object types, each object has a different number of sample data; in the experiment, the average value of all samples of each object is used as the typical spectral feature of the object.
[0057] Such as Figure 4 As shown, the specific implementation steps are:
[0058] (1) Carry out data preprocessing on the original hyperspectral remote sensing image data, remove noise bands, and then determine the number of bands k to be selected, typical spectral data and training sample data.
[0059] (2) Choose B 1 and B 2 As the initial ...
Embodiment 2
[0074] Example 2: The hyperspectral image Cuprite data of band 224 in the Nevada region acquired by the AVIRIS sensor on June 19, 1997, the size of the sub-image is 350×350 pixels, and the spatial resolution of the data is 20m. After removing the water absorption and low SNR bands, 189 bands were retained. The image contains five minerals: Alunite, Buddingtonite, Calcite, Kaolinite and Muscovite. According to field investigation, the image actually contains more than 20 kinds of minerals.
[0075] In order to verify the proposed k-value estimation algorithm, this experiment is designed. According to the existing literature, the VD of the data, that is, the number of end members, is about 22. Therefore, theoretically speaking, when using K-means clustering on this data, its k value should also be around 22. Algorithm RICD, R 2 、SR 2 and the experimental results of pseudoF as Figure 6(a) to Figure 6(d) shown.
[0076] According to the theory of evaluating the number of k...
PUM
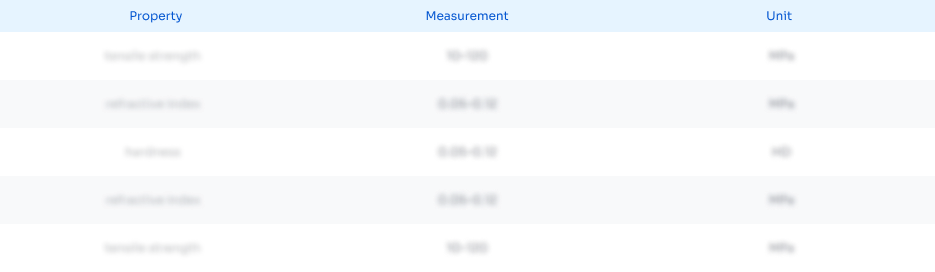
Abstract
Description
Claims
Application Information
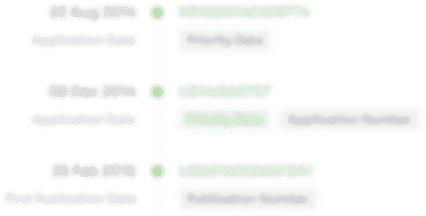
- R&D
- Intellectual Property
- Life Sciences
- Materials
- Tech Scout
- Unparalleled Data Quality
- Higher Quality Content
- 60% Fewer Hallucinations
Browse by: Latest US Patents, China's latest patents, Technical Efficacy Thesaurus, Application Domain, Technology Topic, Popular Technical Reports.
© 2025 PatSnap. All rights reserved.Legal|Privacy policy|Modern Slavery Act Transparency Statement|Sitemap|About US| Contact US: help@patsnap.com