Epidemic propagation network modeling and inference of based on autonomic computing
A technology for spreading networks and epidemics, applied in computing, special data processing applications, instruments, etc., and can solve problems such as difficult to expand models, limited epidemic monitoring data, and difficulties in spreading network inference methods.
- Summary
- Abstract
- Description
- Claims
- Application Information
AI Technical Summary
Problems solved by technology
Method used
Image
Examples
example 1
[0058] Example 1: Model Validation Based on Real Data
[0059] Test the plausibility of the above epidemic propagation network model. The experimental data used the real monitoring data of the 2009 H1N1 swine flu outbreak in Hong Kong, which recorded the changes in the cumulative cases in Hong Kong from May 2009 to March 2010. Figure 4 As shown by the red solid line, H1N1 patients appeared between May 1 and June 12, but large-scale transmission did not begin, and the number of infected people increased rapidly after June 12. In the middle and late stages of the H1N1 outbreak, the Hong Kong government implemented measures such as patient isolation and vaccination to control the spread of H1N1, and the number of new cases gradually decreased. Since the epidemic transmission model provided by the present invention does not consider the impact of human control measures on epidemic transmission, the data of the first three months with less human intervention during the H1N1 outbr...
example 2
[0063] Example 2 Outbreak trend and model parameter estimation
[0064] To test the parameter estimation method of the D-AOC system, the specific steps are as follows: ①Given a set of system parameters θ={A, θ 1 , θ 2 , θ 3 , θ 4 , θ 5}, where θ i =i , gamma i , τ i>, 1≤i≤5; ② Run the D-AOC system to simulate the spread of the epidemic based on θ, and record the change of the number of infected cases over time during this process, as the observed epidemic monitoring data D; ③ Based on D, use image 3 The method in estimates the parameters and epidemic trends ④Comparing the actual value with the estimated value, analyzing the estimated situation of parameters and outbreak trend. Figure 5 As shown in (a), the bar graph represents the epidemic surveillance data D (time series of new cases) obtained through the above step ②, and the red solid line represents the outbreak trend estimated through the above step ③ (time series of new case estimates). Estimated outbrea...
example 3
[0068] Example 3 Impact of Missing Data on Outbreak Trend Estimation
[0069] The actually available monitoring data may be missing to varying degrees. Although the missing data is not visible, the impact on the spread of the epidemic actually exists. After estimating the outbreak trend of the epidemic based on the missing surveillance data, the missing transmission data can be guessed from the estimated data. In this experiment, we study the impact of missing data in different situations on the estimation of the outbreak trend. First, we run the D-AOC system with the parameters in Table 1 to simulate the spread of the epidemic, and use different time scales to sample from it to obtain the observed monitoring data; based on Estimate parameters and outbreak trends from the monitoring data obtained by sampling; according to the formula Calculate the deviation between the estimated outbreak trend and the actual monitoring data, and then analyze the impact of missing data in dif...
PUM
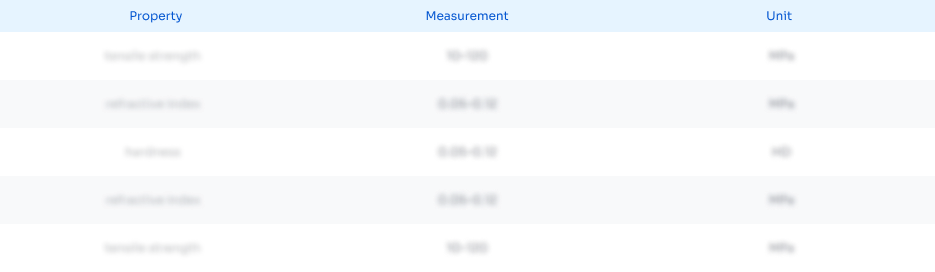
Abstract
Description
Claims
Application Information
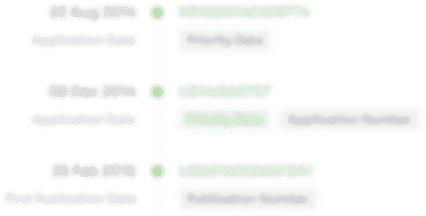
- Generate Ideas
- Intellectual Property
- Life Sciences
- Materials
- Tech Scout
- Unparalleled Data Quality
- Higher Quality Content
- 60% Fewer Hallucinations
Browse by: Latest US Patents, China's latest patents, Technical Efficacy Thesaurus, Application Domain, Technology Topic, Popular Technical Reports.
© 2025 PatSnap. All rights reserved.Legal|Privacy policy|Modern Slavery Act Transparency Statement|Sitemap|About US| Contact US: help@patsnap.com