Online lithium ion battery residual life predicting method based on relevance vector regression
A lithium-ion battery and correlation vector technology, applied in the field of lithium-ion battery life prediction, can solve problems such as low prediction accuracy
- Summary
- Abstract
- Description
- Claims
- Application Information
AI Technical Summary
Problems solved by technology
Method used
Image
Examples
specific Embodiment approach 1
[0044] Specific implementation mode 1: the following combination figure 1 To explain this embodiment, the method for online prediction of the remaining life of a lithium-ion battery based on correlation vector regression in this embodiment includes the following steps:
[0045] Step 1: Select the capacity data IS=(C 1 , C 2 ,...C n ) As the original sample, C i Is the battery capacity, the unit is Ah, i=1, 2,..., n, n is a positive integer;
[0046] Perform phase space reconstruction to construct a training sample set: set the embedding dimension l=5 and delay d=1 to obtain the training sample set {(x 1 , Y 1 ), (x 2 , Y 2 ),..., (x n-l , Y n-l )}, where x j =(C j , C j+1 ,..., C j+l-1 ), y j =C j+l , J = 1, 2, ... n-l, where x = (x 1 , X 2 ,..., x n-l ) Is the input data for the relevance vector machine RVM model, y=(y 1 , Y 2 ,..., y n-l ) Is the output data of the correlation vector machine RVM model;
[0047] Step 2: Initialize the RVM model parameters of the correlation vector m...
specific Embodiment approach 2
[0076] Specific embodiment two: this embodiment is a further description of the first embodiment, and the prediction error limit in this embodiment is PEB=0.1.
specific Embodiment approach 3
[0077] Specific implementation manner three: the following combination Figure 1 to Figure 3 This embodiment is described. This embodiment is a further explanation of the first or second embodiment. In this embodiment, the failure threshold U=1.38Ah.
[0078] Most of the driven lithium-ion battery remaining life prediction methods are offline, with low dynamic update ability and low prediction accuracy, and the calculation efficiency of online retraining is low, especially the correlation vector machine algorithm is directly used for online modeling And forecast. At the same time, due to the sparsity of the relevance vector machine algorithm and the challenges in the long-term prediction of the remaining life, it is difficult for the offline relevance vector machine algorithm to obtain accurate prediction results. Therefore, the update of the sample, the retraining of the model, and the dynamic characteristics of online update with the sample are very important in the remaining l...
PUM
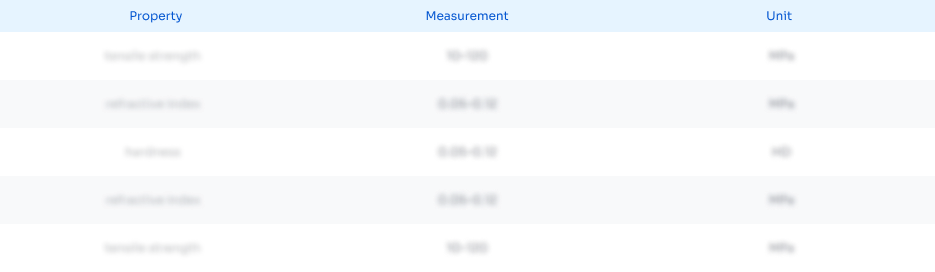
Abstract
Description
Claims
Application Information
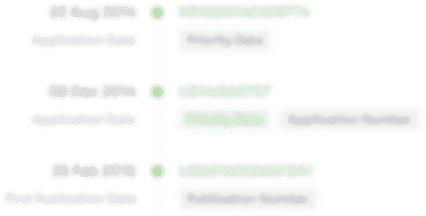
- R&D Engineer
- R&D Manager
- IP Professional
- Industry Leading Data Capabilities
- Powerful AI technology
- Patent DNA Extraction
Browse by: Latest US Patents, China's latest patents, Technical Efficacy Thesaurus, Application Domain, Technology Topic, Popular Technical Reports.
© 2024 PatSnap. All rights reserved.Legal|Privacy policy|Modern Slavery Act Transparency Statement|Sitemap|About US| Contact US: help@patsnap.com