Image de-noising method based on sparse self-adapted dictionary
An adaptive dictionary and image technology, applied in the field of image processing, can solve the problems of slow dictionary learning, noise in the dictionary, affecting the denoising effect, etc., and achieve the effect of improving the peak signal-to-noise ratio, improving PSNR, and good sparsity.
- Summary
- Abstract
- Description
- Claims
- Application Information
AI Technical Summary
Problems solved by technology
Method used
Image
Examples
Embodiment Construction
[0053] Refer to attached figure 1 , the concrete steps of the present invention are as follows:
[0054] Step 1 Obtain a training dataset Y on noisy images:
[0055] Note that the input image is Starting from the first point in the upper left corner of the noise image, scan from top to bottom, from left to right, and take the size of the current point i as the center in turn. The image patch; then columnarize the image patch into a vector y i , and form the training data set Y={y i |i∈1,2,...,N}, where N is the number of image blocks.
[0056] Step 2 uses the dataset Y to train the dictionary:
[0057] 2a) Let the number of iterations J be 15, the number of atoms in the dictionary M=4n, and the initial dictionary D (0) The size of is a discrete cosine dictionary of n×M, and the loop variable k=1;
[0058] 2b) For each column signal y in the data matrix Y i , i∈{1,2,...,N}, solve it in the dictionary D (k-1) Coding coefficient α on i , that is, use the orthogonal ma...
PUM
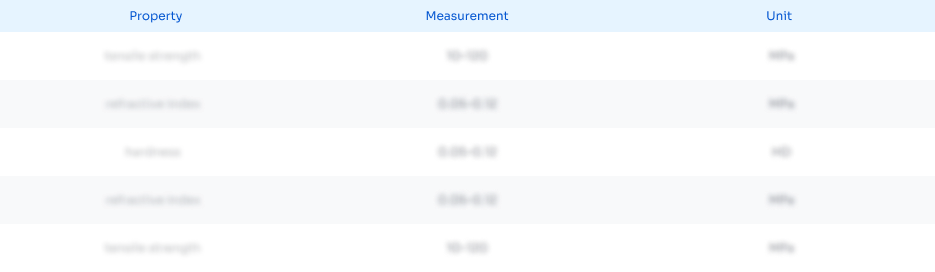
Abstract
Description
Claims
Application Information
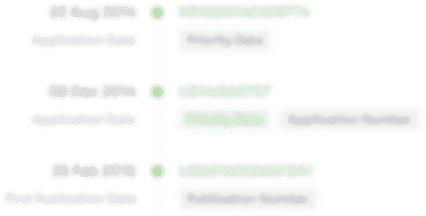
- R&D Engineer
- R&D Manager
- IP Professional
- Industry Leading Data Capabilities
- Powerful AI technology
- Patent DNA Extraction
Browse by: Latest US Patents, China's latest patents, Technical Efficacy Thesaurus, Application Domain, Technology Topic, Popular Technical Reports.
© 2024 PatSnap. All rights reserved.Legal|Privacy policy|Modern Slavery Act Transparency Statement|Sitemap|About US| Contact US: help@patsnap.com