Nonparametric automatic detection method of focal niduses
An automatic detection, non-parametric technology, used in instruments, character and pattern recognition, computer parts and other directions, can solve the problems of reducing the identification ability of local image descriptors, overfitting of parameter models, poor data classification performance, etc. Convenience, stable class estimation, and the effect of accurate detection
- Summary
- Abstract
- Description
- Claims
- Application Information
AI Technical Summary
Problems solved by technology
Method used
Image
Examples
Embodiment 1
[0048] A kind of nonparametric automatic detection method of focal lesion of the present invention, such as figure 1 As shown, the sample set A is constructed in advance by using the medical lesion image that has been delineated as a sample. 1 Database, respectively extract sample set A 1 The local descriptors of the target area and the background area of each image in the image, get the sample set A 1 The target feature library F composed of all target region local descriptors in 1 and sample set A 1 The background feature library F composed of local descriptors of all background regions in 2 .
[0049] Specifically, the sample set A is obtained as follows: 1 The target feature library F 1 and background feature library F 2 :
[0050] (0.1) Define the lesion area in the medical lesion image as the sample as the target area, and the area outside the lesion is the background area, and extract its local descriptor for each pixel in the target area; the same for each p...
Embodiment 2
[0085] The method of the present invention is described with a specific embodiment.
[0086] The database used in this embodiment has 458 liver CT image sample sets, including: 178 liver cancers, 98 liver cysts, and 182 hepatic hemangiomas, and each CT image has been manually delineated.
PUM
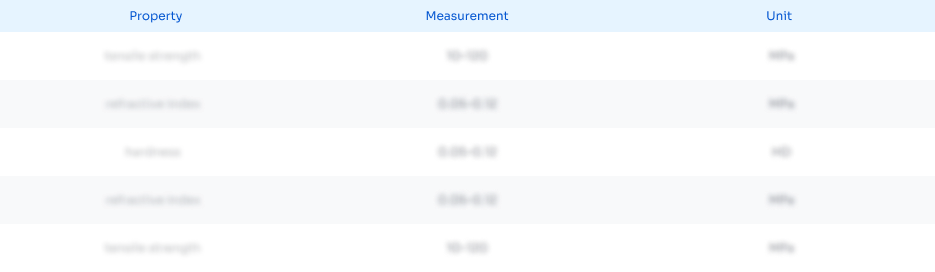
Abstract
Description
Claims
Application Information
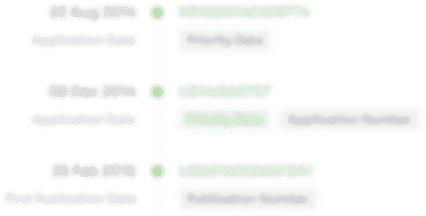
- Generate Ideas
- Intellectual Property
- Life Sciences
- Materials
- Tech Scout
- Unparalleled Data Quality
- Higher Quality Content
- 60% Fewer Hallucinations
Browse by: Latest US Patents, China's latest patents, Technical Efficacy Thesaurus, Application Domain, Technology Topic, Popular Technical Reports.
© 2025 PatSnap. All rights reserved.Legal|Privacy policy|Modern Slavery Act Transparency Statement|Sitemap|About US| Contact US: help@patsnap.com