Topic modeling method based on selected cell
A technology for topic modeling and selection of units, applied in special data processing applications, instruments, electrical digital data processing, etc., can solve the problem of not considering words to express other topics or noise
- Summary
- Abstract
- Description
- Claims
- Application Information
AI Technical Summary
Problems solved by technology
Method used
Image
Examples
Embodiment 1
[0106] Taking the text type query "NYT+CNN" submitted by the user as an example, the steps of the present invention to process the query in the database are as follows:
[0107] 1. Search the multimedia database for all the news published by NYT and CNN, and extract the text in the search results;
[0108] 2. Use natural language processing tools to divide the document into sentences, and use the obtained sentences as the fragment structure of the data;
[0109] 3. Use natural language processing tools to mark the part-of-speech of each word, and use the obtained part-of-speech tagging structure as the feature of each word;
[0110] 4. Remove useless high-frequency words and uncommon words with low frequency;
[0111] 5. Collect all the words that have appeared in the text after statistical processing to form a vocabulary.
[0112] 6. According to the data set covered by the data, determine the number of topics to be 20;
[0113] 7. For each sentence contained in the data s...
Embodiment 2
[0140] Taking the image type query "LabelMe+MSRC" submitted by the user as an example, the steps of the present invention to process the query in the database are as follows:
[0141] 1. Two image data sets, LabelMe and MSRC v2, were found in the multimedia database, and the images in the search results were extracted;
[0142] 2. Use OpenSIFT to extract the SIFT features of all pictures to form a set of 128-dimensional feature points;
[0143] 3. Use K-means to cluster the feature point set to obtain a set of visual dictionaries, and replace all SIFT point clustering results with visual words in the dictionary;
[0144] 4. Use the existing annotations to extract attributes such as object boundaries and color histograms in the image, and use the object boundaries as the fragment structure in the image;
[0145] 5. The objects are clustered to obtain the category label to which each visual word belongs, and the category label is used as the feature of the visual word.
[0146...
PUM
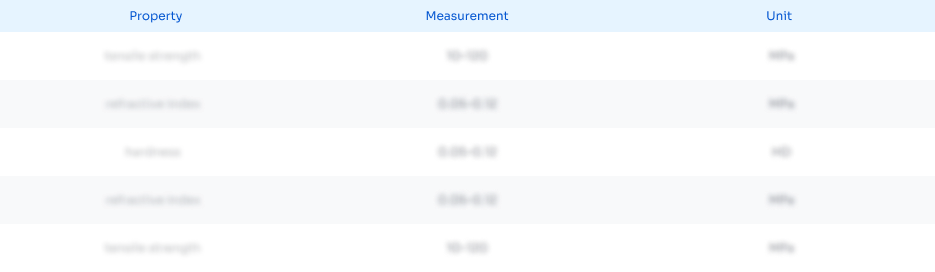
Abstract
Description
Claims
Application Information
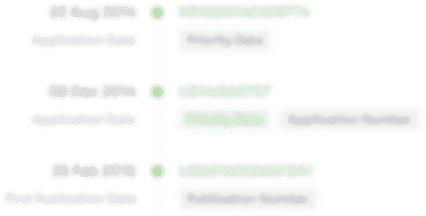
- R&D Engineer
- R&D Manager
- IP Professional
- Industry Leading Data Capabilities
- Powerful AI technology
- Patent DNA Extraction
Browse by: Latest US Patents, China's latest patents, Technical Efficacy Thesaurus, Application Domain, Technology Topic, Popular Technical Reports.
© 2024 PatSnap. All rights reserved.Legal|Privacy policy|Modern Slavery Act Transparency Statement|Sitemap|About US| Contact US: help@patsnap.com