WiFi indoor positioning method of semi-supervised manifold learning based on category matching
A manifold learning and indoor positioning technology, applied in the WiFi indoor positioning field of semi-supervised manifold learning, can solve the problems of difficult mobile terminal implementation, difficult real-time requirements, and difficult to obtain.
- Summary
- Abstract
- Description
- Claims
- Application Information
AI Technical Summary
Problems solved by technology
Method used
Image
Examples
specific Embodiment approach 1
[0040] Specific implementation mode one: The offline stage positioning process of the WiFi indoor positioning method based on category matching semi-supervised manifold learning in this embodiment is implemented in the following steps:
[0041] 1. Arrange APs in the indoor area to be positioned, so that the wireless signal covers the indoor area to be positioned, and complete the construction of the WiFi network;
[0042] Select and record the corresponding coordinates of the reference point in the indoor area to be positioned, measure and record the RSS signals of all APs received by the reference point in turn as location feature information, construct a Radio Map, and store the Radio Map;
[0043] 2. Use the GMST eigendimension estimation algorithm to analyze the eigendimension of the Radio Map constructed in step 1. The obtained eigendimension is used as one of the input parameters of the CM-SDE algorithm to determine the dimensionality of the Radio Map after dimension redu...
specific Embodiment approach 2
[0054] Specific embodiment two: the difference between this embodiment and specific embodiment one is: adopt GMST intrinsic dimension estimation algorithm to analyze the intrinsic dimension of the Radio Map constructed in step one in step two, its calculation formula is:
[0055] Geodesic minimum spanning tree algorithm
[0056] In the above formula A in represents the slope of the linear fitting expression y=ax+b of the minimum spanning tree.
[0057] Other steps and parameters are the same as those in Embodiment 1.
specific Embodiment approach 3
[0058] Specific embodiment three: the difference between this embodiment and specific embodiment one or two is: in step four, generate a new dimensionality-reduced signal coverage map RadioMap * And the V' expression in step 6 is:
[0059] Radio Map*=V′·X
[0060] X is a Radio Map that needs dimensionality reduction.
[0061] Other steps and parameters are the same as those in Embodiment 1 or Embodiment 2.
PUM
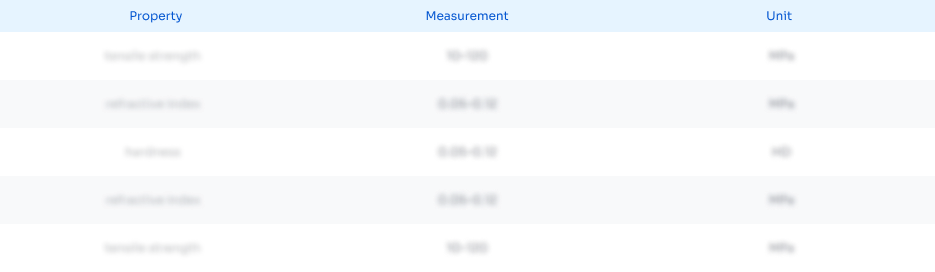
Abstract
Description
Claims
Application Information
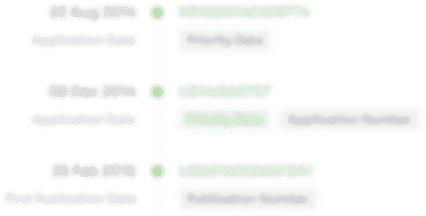
- R&D Engineer
- R&D Manager
- IP Professional
- Industry Leading Data Capabilities
- Powerful AI technology
- Patent DNA Extraction
Browse by: Latest US Patents, China's latest patents, Technical Efficacy Thesaurus, Application Domain, Technology Topic.
© 2024 PatSnap. All rights reserved.Legal|Privacy policy|Modern Slavery Act Transparency Statement|Sitemap