Sorting method based on non-supervision feature selection
A feature selection and classification method technology, applied in the field of data processing, can solve the problems of not being able to generate feature subsets, ignoring associations, and not being able to obtain classification results, so as to achieve the effect of improving classification speed and classification accuracy
- Summary
- Abstract
- Description
- Claims
- Application Information
AI Technical Summary
Problems solved by technology
Method used
Image
Examples
Embodiment Construction
[0029] Aiming at the impact of the "curse of dimensionality" on high-dimensional data mining, the present invention first obtains the similarity matrix of high-dimensional data through spectrogram theory and ITML metric learning, and then uses the SM algorithm to complete the mapping from the original sample set to the feature vector space. Coefficient vectors and MCFS scores for feature selection. Finally, the support vector machine is used to establish a classification model for the data after feature selection and classify the driver's EEG data to verify the effectiveness of the algorithm. Compared with other algorithms, the present invention well preserves the correlation between high-dimensional data features when performing feature selection before building a classification model, and is beneficial to overcome the impact of the "curse of dimensionality" on high-dimensional data.
[0030] Such as figure 1 , figure 2 Shown, the present invention is based on the classifi...
PUM
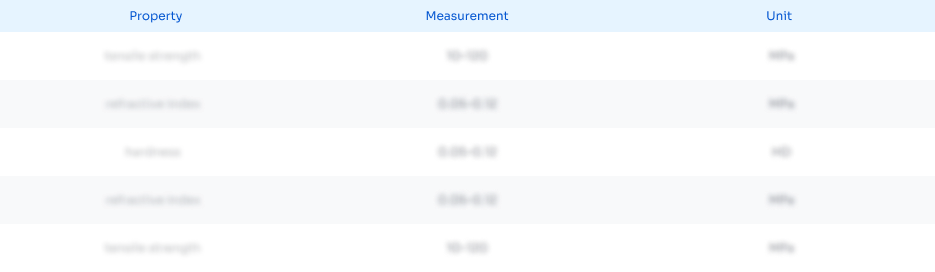
Abstract
Description
Claims
Application Information
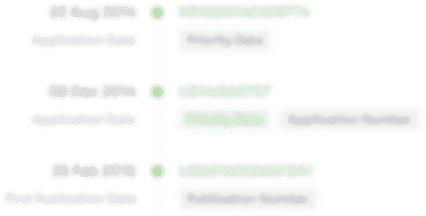
- R&D Engineer
- R&D Manager
- IP Professional
- Industry Leading Data Capabilities
- Powerful AI technology
- Patent DNA Extraction
Browse by: Latest US Patents, China's latest patents, Technical Efficacy Thesaurus, Application Domain, Technology Topic, Popular Technical Reports.
© 2024 PatSnap. All rights reserved.Legal|Privacy policy|Modern Slavery Act Transparency Statement|Sitemap|About US| Contact US: help@patsnap.com