Valueless image removing method based on deep convolutional neural networks
A neural network and deep convolution technology, applied in the field of worthless image removal based on deep convolutional neural network, can solve problems such as limitations, poor noise resistance, and inability to fully express images.
- Summary
- Abstract
- Description
- Claims
- Application Information
AI Technical Summary
Problems solved by technology
Method used
Image
Examples
Embodiment Construction
[0044] The technical solution adopted by the present invention to solve the technical problem is: a method for removing worthless images based on a deep convolutional neural network.
[0045] The technical solution of the present invention includes the following steps: pre-training based on sparse autoencoder, constructing deep convolutional neural network and training softmax classification model.
[0046] (a) Perform whitening preprocessing on the aerial image training set. Since there is a strong correlation between adjacent pixels in the image, the purpose of whitening is to reduce the redundancy of the input. Before whitening the data, the feature zero mean should be performed. Select appropriate regularization parameters to smooth the input image and eliminate noise;
[0047] The sparse autoencoder network is an unsupervised learning algorithm. The image training set after whitening preprocessing is input into the sparse autoencoder network, and the partial derivative ex...
PUM
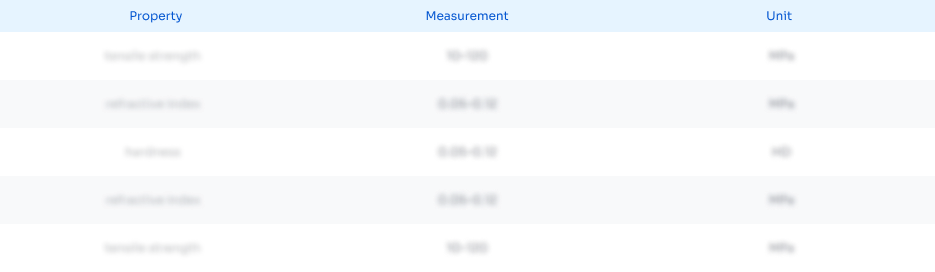
Abstract
Description
Claims
Application Information
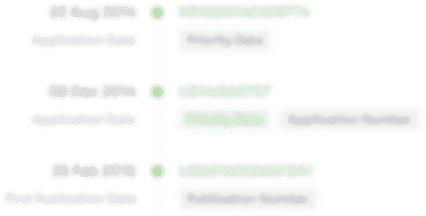
- R&D Engineer
- R&D Manager
- IP Professional
- Industry Leading Data Capabilities
- Powerful AI technology
- Patent DNA Extraction
Browse by: Latest US Patents, China's latest patents, Technical Efficacy Thesaurus, Application Domain, Technology Topic.
© 2024 PatSnap. All rights reserved.Legal|Privacy policy|Modern Slavery Act Transparency Statement|Sitemap