Configurable convolutional neural network based red green blue-distance (RGB-D) figure behavior identification method
A convolutional neural network, RGB-D technology, applied in the field of RGB-D character behavior recognition based on configurable convolutional neural network, can solve the problem of low accuracy, difficulty in expressing sub-action time changes, and difficult character recognition and other issues, to achieve the effect of high accuracy
- Summary
- Abstract
- Description
- Claims
- Application Information
AI Technical Summary
Problems solved by technology
Method used
Image
Examples
Embodiment Construction
[0030] The present invention will be further described below in conjunction with the accompanying drawings, but the embodiments of the present invention are not limited thereto.
[0031] 1. Structured deep model
[0032] First, the structured deep model and the introduced hidden variables are introduced in detail.
[0033] 1.1 Deep Convolutional Neural Network
[0034] In order to model complex character behaviors, the depth model in this embodiment is as follows image 3 shown. It consists of M subnetworks and two fully connected layers. Among them, the outputs of M subnetworks are concatenated into a long vector, and then connected with two fully connected layers. ( image 3 where M is 3, and each sub-network is represented by a different pattern) Each sub-network processes its corresponding video segment, which is related to a sub-behavior decomposed from the complex behavior. Each sub-network is sequentially composed of a 3D convolutional layer, a downsampling layer,...
PUM
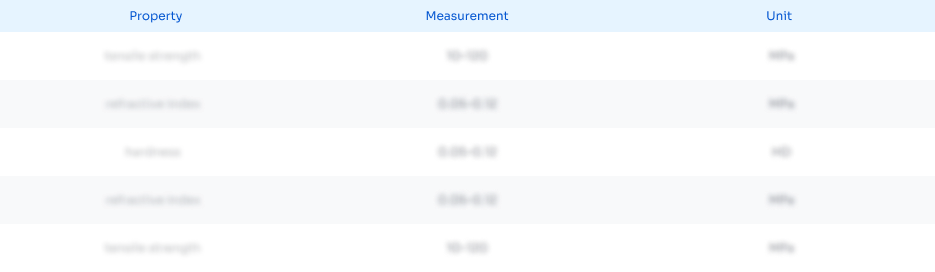
Abstract
Description
Claims
Application Information
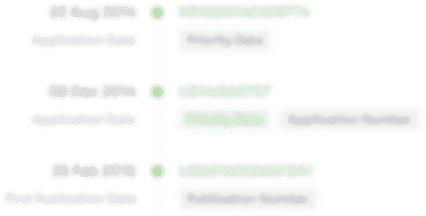
- R&D
- Intellectual Property
- Life Sciences
- Materials
- Tech Scout
- Unparalleled Data Quality
- Higher Quality Content
- 60% Fewer Hallucinations
Browse by: Latest US Patents, China's latest patents, Technical Efficacy Thesaurus, Application Domain, Technology Topic, Popular Technical Reports.
© 2025 PatSnap. All rights reserved.Legal|Privacy policy|Modern Slavery Act Transparency Statement|Sitemap|About US| Contact US: help@patsnap.com