Hyperspectral image classification method based on semi-supervised dictionary learning
A hyperspectral image and dictionary learning technology, applied in the field of semi-supervised learning and sparse representation, hyperspectral image classification, can solve the problems of SVM classification performance impact and inability to effectively improve classification accuracy
- Summary
- Abstract
- Description
- Claims
- Application Information
AI Technical Summary
Problems solved by technology
Method used
Image
Examples
Embodiment Construction
[0047] refer to figure 1 , the specific implementation steps of the present invention include:
[0048] Step 1. Input a hyperspectral image I, which contains n pixels of type c ground objects. Each pixel is used as a sample. Each sample is represented by a spectral feature vector, and the feature dimension of the sample is d.
[0049] Step 2, construct a labeled sample set X L , class label set Y L , unlabeled sample set X U and the test sample set X T .
[0050] 2a) Randomly select an equal number of samples from each type of ground object pixel as marked samples, a total of nl marked samples constitute a marked sample set in, Indicates the i-th sample of the labeled sample set; the corresponding class label set of the labeled sample set is in, is the class label of the i-th sample in the labeled sample set; R d Represents a d-dimensional vector space;
[0051] 2b) Randomly select n from samples other than the labeled sample set u samples are used as unlabeled ...
PUM
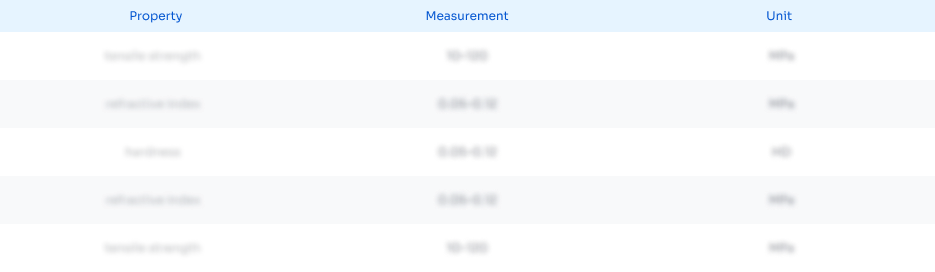
Abstract
Description
Claims
Application Information
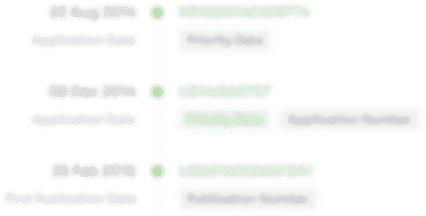
- R&D
- Intellectual Property
- Life Sciences
- Materials
- Tech Scout
- Unparalleled Data Quality
- Higher Quality Content
- 60% Fewer Hallucinations
Browse by: Latest US Patents, China's latest patents, Technical Efficacy Thesaurus, Application Domain, Technology Topic, Popular Technical Reports.
© 2025 PatSnap. All rights reserved.Legal|Privacy policy|Modern Slavery Act Transparency Statement|Sitemap|About US| Contact US: help@patsnap.com