Driver-vehicle classification method and device
A classification method and inter-class difference technology, which is applied in the direction of neural learning methods, instruments, biological neural network models, etc., can solve problems that affect accuracy, expand the number of layers, affect speed, etc., and achieve a balance between speed and accuracy, Good balance between speed and precision
- Summary
- Abstract
- Description
- Claims
- Application Information
AI Technical Summary
Problems solved by technology
Method used
Image
Examples
Embodiment 1
[0026] A very important aspect of the human-vehicle classification method based on the deep convolutional neural network is the expansion of the number of neural network layers. If the number of convolutional layers is expanded too much, the speed of running the human-vehicle classification method will slow down and affect the work. Efficiency; if the number of convolutional layers is expanded too little, the calculation accuracy will be reduced, affecting the quality of work. In practical applications, the number of convolutional layers is usually extended to 3, 4 or 5 layers. The increase in the number of convolutional layers is generally beneficial to the improvement of accuracy, but it will slow down the operation speed. How to balance the speed and accuracy has a great impact on the realization of the method. In actual work, the expansion of the number of layers in the traditional way may not be the most suitable choice. For example, when the number of layers is expanded ...
Embodiment 2
[0100] The device for classifying people and vehicles in this embodiment includes two modules: a training module and a classification module. The following describes in detail how the two modules work during a process of classifying people and vehicles.
[0101] The training module is used to read the training sample set offline, and use the training sample set to expand the number of convolutional layers of the deep convolutional neural network, as shown in equations (4) and (5), to calculate the intra-class difference of the training sample set s1 and inter-class difference s2, calculate the ratio of s1 and s2, the ratio of s1 and s2 is the difference ratio s1 / s2. As shown in formula (6), the difference ratio is compared with the threshold, and the number of convolutional layers N of the deep convolutional neural network is adaptively expanded according to the comparison result. The threshold includes the first threshold d1 and the second threshold d2, which is Obtained thro...
Embodiment 3
[0110] The method for classifying people and vehicles in this embodiment is divided into two processes: a training process and a classification process. The detailed steps of each process are as follows.
[0111] Such as Figure 6 Shown is the flow chart of the training process, and the following content describes the specific steps of the training process in detail. Receive image samples from the training sample set, and set the learning rate and number of iterations of the BP algorithm. Use the training sample set to expand the number of convolutional layers of the deep convolutional neural network, as shown in equations (4) and (5), calculate the intra-class difference s1 and the inter-class difference s2 of the training sample set, and calculate s1 and The ratio of s2, the ratio of s1 and s2 is the difference ratio s1 / s2. As shown in formula (6), the difference ratio is compared with the threshold, and the number of convolutional layers N of the deep convolutional neural...
PUM
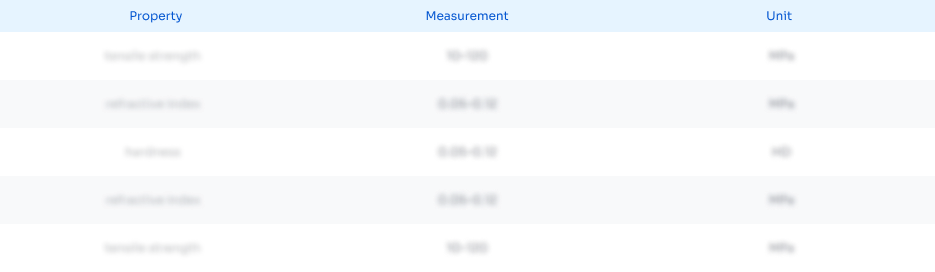
Abstract
Description
Claims
Application Information
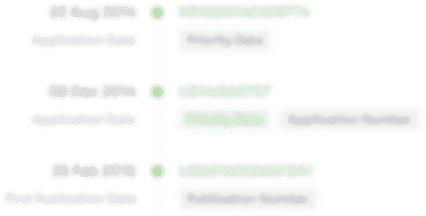
- R&D Engineer
- R&D Manager
- IP Professional
- Industry Leading Data Capabilities
- Powerful AI technology
- Patent DNA Extraction
Browse by: Latest US Patents, China's latest patents, Technical Efficacy Thesaurus, Application Domain, Technology Topic, Popular Technical Reports.
© 2024 PatSnap. All rights reserved.Legal|Privacy policy|Modern Slavery Act Transparency Statement|Sitemap|About US| Contact US: help@patsnap.com