Remote sensing image classification method based on active deep learning
A technology of remote sensing images and classification methods, which is applied in the field of remote sensing image classification based on active deep learning, can solve problems such as imbalanced data learning problems, and achieve the effects of reducing complexity, improving efficiency, and reducing the number of samples
- Summary
- Abstract
- Description
- Claims
- Application Information
AI Technical Summary
Problems solved by technology
Method used
Image
Examples
Embodiment Construction
[0020] The following will clearly and completely describe the technical solutions in the embodiments of the present invention with reference to the accompanying drawings in the embodiments of the present invention. Obviously, the described embodiments are only some, not all, embodiments of the present invention. All other embodiments obtained by persons of ordinary skill in the art based on the embodiments of the present invention belong to the protection scope of the present invention.
[0021] Such as figure 1 As shown, a remote sensing image classification method based on active deep learning according to an embodiment of the present invention includes the following steps:
[0022] Step 1: Select the remote sensing image data to be classified;
[0023] Step 2: Using a pre-configured algorithm to process the remote sensing image data, the specific steps include:
[0024] Step 2-1: Use all training samples of remote sensing image data for unsupervised self-encoding deep net...
PUM
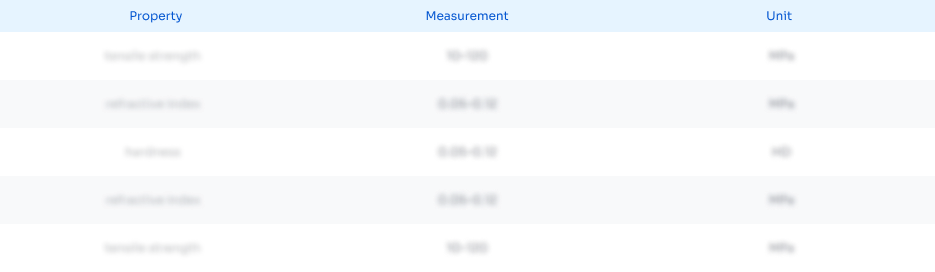
Abstract
Description
Claims
Application Information
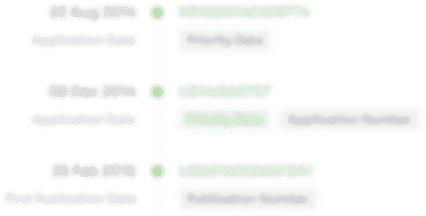
- R&D Engineer
- R&D Manager
- IP Professional
- Industry Leading Data Capabilities
- Powerful AI technology
- Patent DNA Extraction
Browse by: Latest US Patents, China's latest patents, Technical Efficacy Thesaurus, Application Domain, Technology Topic, Popular Technical Reports.
© 2024 PatSnap. All rights reserved.Legal|Privacy policy|Modern Slavery Act Transparency Statement|Sitemap|About US| Contact US: help@patsnap.com