Image sequence category labeling method based on mixed graph model
A technology of image sequence and mixed graph, applied in the field of computer vision, can solve problems such as image sequence labeling that has not yet been applied
- Summary
- Abstract
- Description
- Claims
- Application Information
AI Technical Summary
Problems solved by technology
Method used
Image
Examples
Embodiment 1
[0119] Treat labeled image sequences figure 1 (a) The simple graph model and the method of the present invention are respectively used for processing. It can be seen from the result comparison diagram that the method of the present invention effectively improves the accuracy and timing consistency of sequence image category labeling.
Embodiment 2
[0121] Image to be detected figure 2 (a) The simple graph model and the method of the present invention are respectively used for processing. It can be seen from the result comparison diagram that the method of the present invention effectively improves the accuracy and timing consistency of sequence image category labeling.
[0122] Table 1 is the accuracy rate (Prec.) on the KITTI data set by using the simple graph model and the method of the present invention, the recall rate (Rec.), the F standard (FM) evaluation numerical results, the higher the value, it shows that the method obtains The labeling results are better. It can be seen from Table 1 that the method of the present invention achieves better results in quantitative indicators than the simple graphical model method.
[0123] Table 1 Comparison data of image sequence experiment results
[0124]
PUM
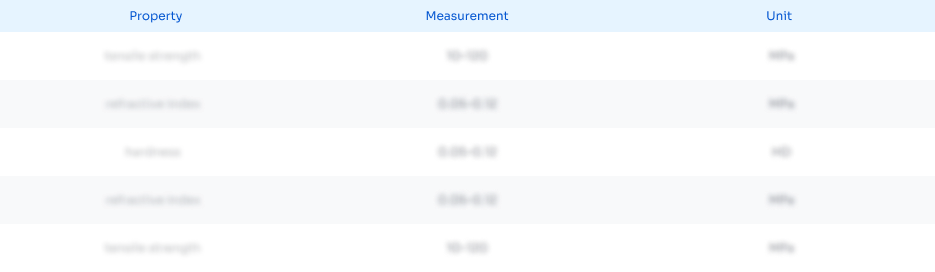
Abstract
Description
Claims
Application Information
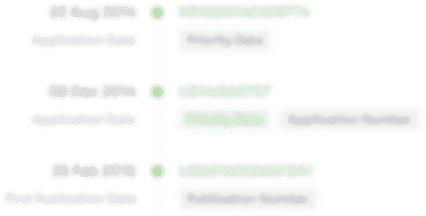
- R&D Engineer
- R&D Manager
- IP Professional
- Industry Leading Data Capabilities
- Powerful AI technology
- Patent DNA Extraction
Browse by: Latest US Patents, China's latest patents, Technical Efficacy Thesaurus, Application Domain, Technology Topic.
© 2024 PatSnap. All rights reserved.Legal|Privacy policy|Modern Slavery Act Transparency Statement|Sitemap