Power maximum load small-sample prediction method
A technology of maximum load and prediction method, applied in the field of power transmission and distribution, it can solve the problems that the prediction accuracy depends on parameters, there is no prediction method, and the LSSVM learning and generalization ability have a great influence.
- Summary
- Abstract
- Description
- Claims
- Application Information
AI Technical Summary
Problems solved by technology
Method used
Image
Examples
Embodiment Construction
[0044] The invention discloses a small-sample forecasting method for the maximum power load, which can carry out LSSVM modeling based on limited small-sample data, and determine the optimum of the kernel function width σ and error penalty factor C of the model through a quantum harmony optimization algorithm values to enable intelligent selection of model parameters while significantly improving prediction accuracy. This forecasting method can not only be applied to the field of maximum load forecasting, but also can solve other forecasting problems.
[0045] Features of the present invention:
[0046] First of all, the present invention uses the idea of quantum harmony search optimization algorithm to conduct a global search, and can find the value of the LSSVM parameter to be optimized corresponding to the minimum objective function, so as to realize the intelligent optimization of the two parameters of the kernel function width σ and the error penalty factor C Select, ...
PUM
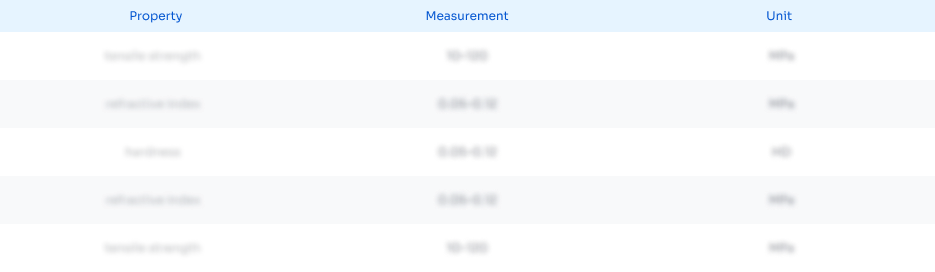
Abstract
Description
Claims
Application Information
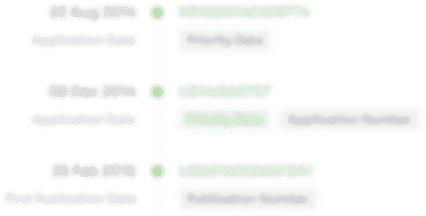
- R&D Engineer
- R&D Manager
- IP Professional
- Industry Leading Data Capabilities
- Powerful AI technology
- Patent DNA Extraction
Browse by: Latest US Patents, China's latest patents, Technical Efficacy Thesaurus, Application Domain, Technology Topic, Popular Technical Reports.
© 2024 PatSnap. All rights reserved.Legal|Privacy policy|Modern Slavery Act Transparency Statement|Sitemap|About US| Contact US: help@patsnap.com