Short period load prediction method based on kernel principle component analysis and random forest
A technology of nuclear principal component analysis and short-term load forecasting, which is applied in the field of power systems, can solve problems such as large amount of calculation, many parameters, and over-fitting, and achieve the effects of controllable generalization error, high prediction accuracy, and few adjustment parameters
- Summary
- Abstract
- Description
- Claims
- Application Information
AI Technical Summary
Problems solved by technology
Method used
Image
Examples
Embodiment Construction
[0023] The technical solution of the present invention will be described in detail below in conjunction with the accompanying drawings. It should be noted that the description here only takes short-term load forecasting as an example, and the invention is also applicable to other fields and fields such as wind speed forecasting and photovoltaic output forecasting.
[0024] In order to improve the prediction accuracy and operational efficiency of short-term load forecasting, the present invention proposes a short-term load forecasting method based on kernel principal component analysis and random forest, such as figure 1 shown. On the one hand, kernel principal component analysis is introduced to perform nonlinear dimensionality reduction on the initial high-dimensional sample input. While ensuring that the input data is relatively small, it retains most of the effective information, reduces the scale of the model, and shortens the running time of the model; On the other hand,...
PUM
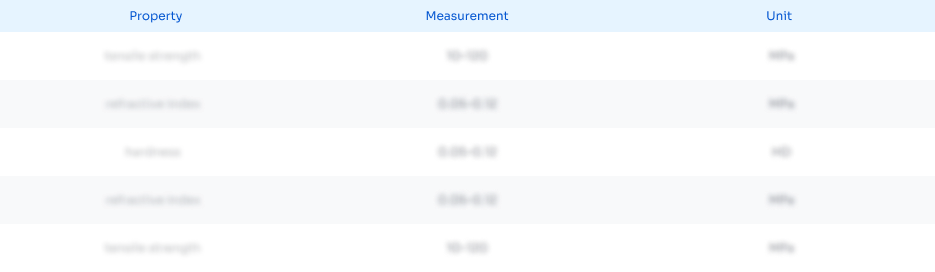
Abstract
Description
Claims
Application Information
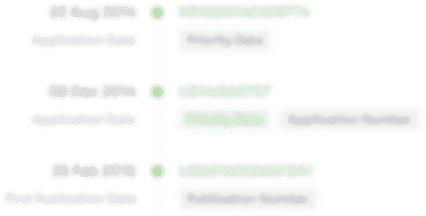
- R&D Engineer
- R&D Manager
- IP Professional
- Industry Leading Data Capabilities
- Powerful AI technology
- Patent DNA Extraction
Browse by: Latest US Patents, China's latest patents, Technical Efficacy Thesaurus, Application Domain, Technology Topic, Popular Technical Reports.
© 2024 PatSnap. All rights reserved.Legal|Privacy policy|Modern Slavery Act Transparency Statement|Sitemap|About US| Contact US: help@patsnap.com