Data Processing Method Based on Incremental Convex Local Nonnegative Matrix Factorization
A non-negative matrix decomposition and data processing technology, which is applied in the field of data processing, can solve the problems of low efficiency of incremental data, consumption of computing resources and storage resources, and inability to handle negative data, so as to save processing time and achieve good positive results. Interaction and sparsity, the effect of improving efficiency
- Summary
- Abstract
- Description
- Claims
- Application Information
AI Technical Summary
Problems solved by technology
Method used
Image
Examples
Embodiment approach
[0040] refer to figure 1 , the present invention is based on the data processing method of incremental convex local non-negative matrix factorization, and its realization steps are as follows:
[0041] Step 1. Obtain data of fixed size p×n as initial data and express it as initial matrix V 0 .
[0042] When processing incremental data, taking image data as an example, each image is arranged column by column into a column vector with dimension p, and finally the data is saved in matrix form, and n samples are selected in order to form the initial matrix V 0 , as the initial data in the data processing process, V 0 The dimension size of is p×n.
[0043] Step 2: Kernel expansion is performed on the objective function of the basic NMF to form an objective function of the convex NMF CNMF.
[0044] Since the basic non-negative matrix factorization NMF cannot directly process the data containing negative elements, the kernel extension can realize the factorization processing of t...
PUM
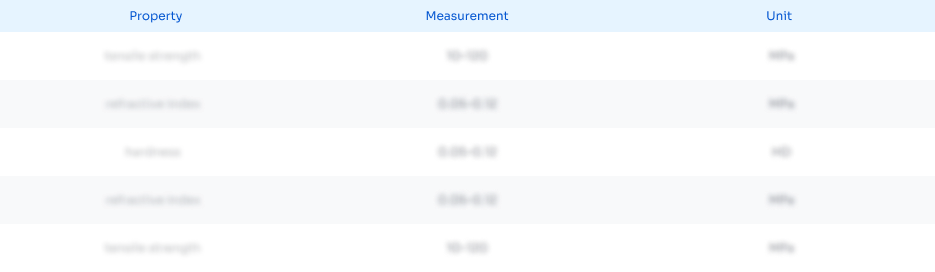
Abstract
Description
Claims
Application Information
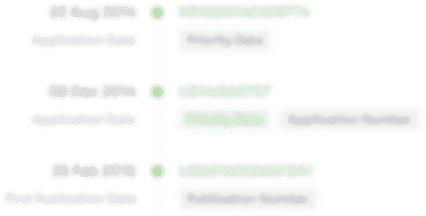
- R&D Engineer
- R&D Manager
- IP Professional
- Industry Leading Data Capabilities
- Powerful AI technology
- Patent DNA Extraction
Browse by: Latest US Patents, China's latest patents, Technical Efficacy Thesaurus, Application Domain, Technology Topic.
© 2024 PatSnap. All rights reserved.Legal|Privacy policy|Modern Slavery Act Transparency Statement|Sitemap