Aluminum electrolytic multi-parameter control method based on BP neural network and MBFO algorithm
A technology of BP neural network and control method, which is applied in the field of multi-parameter control of aluminum electrolysis based on BP neural network and MBFO algorithm, can solve the problems of nonlinearity, low efficiency, and a large amount of greenhouse gases, so as to achieve rapid acquisition and improve production efficiency. Effect
- Summary
- Abstract
- Description
- Claims
- Application Information
AI Technical Summary
Problems solved by technology
Method used
Image
Examples
Example Embodiment
[0042] The present invention will be further described below in conjunction with the embodiments and drawings.
[0043] Such as figure 1 The key to the multi-parameter control method of aluminum electrolysis based on BP neural network and MBFO algorithm is that it includes the following steps:
[0044] S1: Select control parameters that have an impact on current efficiency, energy consumption per ton of aluminum and perfluoride emissions to form decision variables X = [x 1 ,x 2 ,...X M ], M is the number of selected parameters;
[0045] Through statistics of the original variables that have an impact on current efficiency, energy consumption per ton of aluminum and perfluoride emissions in the aluminum electrolysis production process, and determine the parameters that have the greatest impact on current efficiency, energy consumption per ton of aluminum and perfluoride emissions as decision-making Variable X;
[0046] Through statistics of the measured parameters in the actual indust...
PUM
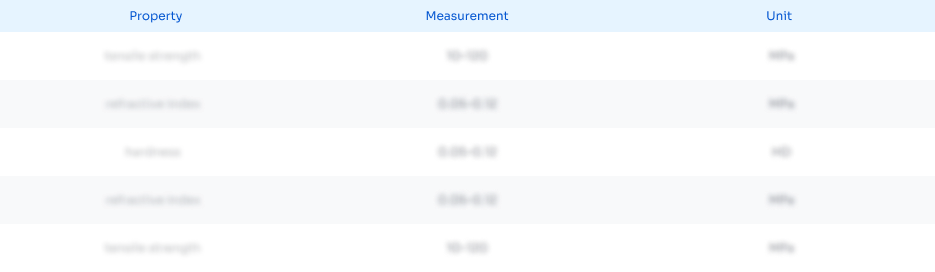
Abstract
Description
Claims
Application Information
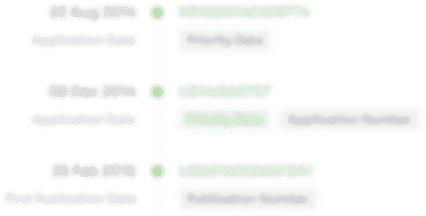
- R&D Engineer
- R&D Manager
- IP Professional
- Industry Leading Data Capabilities
- Powerful AI technology
- Patent DNA Extraction
Browse by: Latest US Patents, China's latest patents, Technical Efficacy Thesaurus, Application Domain, Technology Topic.
© 2024 PatSnap. All rights reserved.Legal|Privacy policy|Modern Slavery Act Transparency Statement|Sitemap