Industrial process fault detection method based on autoregression dynamic hidden variable model
A fault detection, industrial process technology, applied in program control, electrical testing/monitoring, testing/monitoring control systems, etc., can solve the problems of ignoring the static characteristics of the internal structure of the data, unfavorable for the implementation of industrial process automation, and low accuracy.
- Summary
- Abstract
- Description
- Claims
- Application Information
AI Technical Summary
Problems solved by technology
Method used
Image
Examples
Embodiment 1
[0061] An industrial process fault detection method based on the autoregressive dynamic hidden variable model, the method aims at the fault detection problem of the industrial process, first uses the distributed control system to collect data under normal working conditions, and establishes the autoregressive dynamic hidden variable model; the model structure The parameters of the model are estimated by the Kalman filter, the Karl smoother and the expectation-maximization algorithm. On this basis, two monitoring statistics T are constructed using the dynamic and static noise of the model 2 and SPE and their corresponding statistical limits and SPE lim ; To monitor the new process data, the Kalman filter can be used to derive the dynamic hidden variables of the test data, and calculate the corresponding statistics and fault detection results.
[0062] The main steps of the technical solution adopted in the present invention are as follows:
[0063] (1): Utilize the data coll...
Embodiment 2
[0119] The effectiveness of the present invention is illustrated below in conjunction with the example of a specific industrial process. The data of this process comes from the US TE (TennesseeEastman--Tennessee-Eastman) chemical process experiment, and the prototype is an actual process flow of Eastman Chemical Company. The data of the TE process has complex characteristics such as high dimensionality, nonlinearity, autocorrelation, and time variation, and has become a common simulation platform in the field of industrial process control and monitoring. The entire TE process includes 41 measured variables and 12 manipulated variables (control variables), of which 41 measured variables include 22 continuous measured variables and 19 component measured values, which are sampled every 3 minutes, including 21 batches of fault data . Of these failures, 16 are known and 5 are unknown1301. Faults 1 to 7 are related to step changes in process variables, such as the inlet temperatur...
PUM
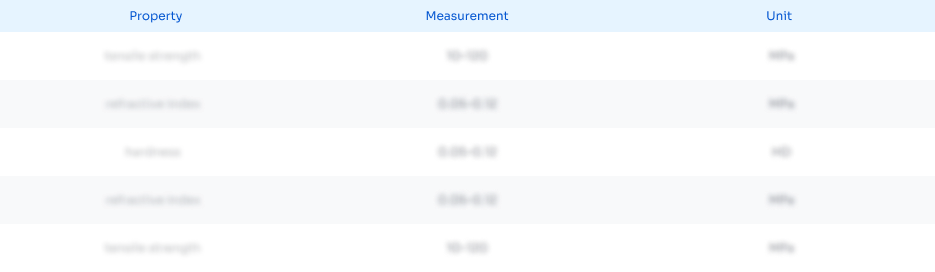
Abstract
Description
Claims
Application Information
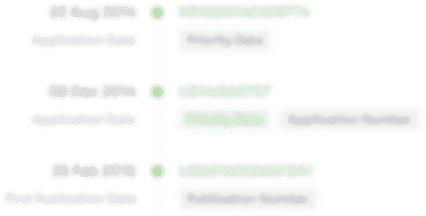
- R&D Engineer
- R&D Manager
- IP Professional
- Industry Leading Data Capabilities
- Powerful AI technology
- Patent DNA Extraction
Browse by: Latest US Patents, China's latest patents, Technical Efficacy Thesaurus, Application Domain, Technology Topic.
© 2024 PatSnap. All rights reserved.Legal|Privacy policy|Modern Slavery Act Transparency Statement|Sitemap