Support vector machine based surface electromyogram signal multi-hand action identification method
A technology of support vector machine and recognition method, which is applied in the field of multi-type hand motion recognition of surface electromyography signals, and can solve the problems of increasing computing load and decreasing model promotion ability.
- Summary
- Abstract
- Description
- Claims
- Application Information
AI Technical Summary
Problems solved by technology
Method used
Image
Examples
Embodiment
[0124] Step (1) The present invention uses the open-source NinaPro data set as the source of myoelectric data, and selects 5 wrist movements, 8 hand postures, and 12 finger movements in the NinaPro data set 1, data of a total of 25 types of gestures. Gesture references involved in the present invention figure 1 .
[0125] Step (2) smoothing and filtering the original data with a window of 50ms, and sampling according to sliding windows of four lengths: 100ms, 150ms, 200ms, and 250ms, and the moving steps of the sliding windows are all 25% of the window length.
PUM
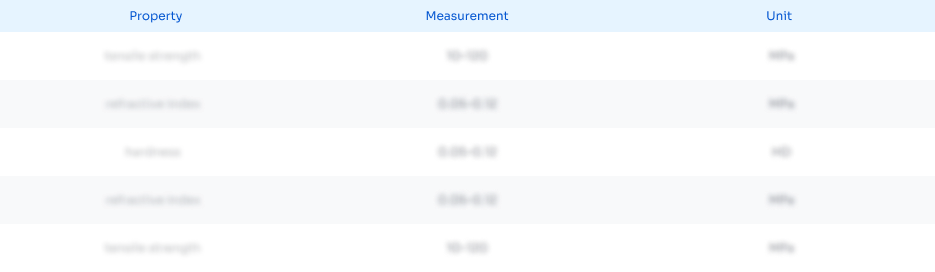
Abstract
Description
Claims
Application Information
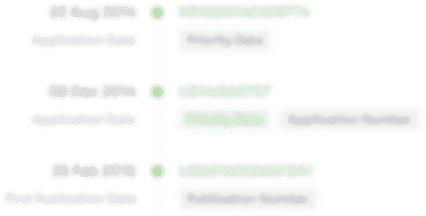
- R&D Engineer
- R&D Manager
- IP Professional
- Industry Leading Data Capabilities
- Powerful AI technology
- Patent DNA Extraction
Browse by: Latest US Patents, China's latest patents, Technical Efficacy Thesaurus, Application Domain, Technology Topic, Popular Technical Reports.
© 2024 PatSnap. All rights reserved.Legal|Privacy policy|Modern Slavery Act Transparency Statement|Sitemap|About US| Contact US: help@patsnap.com