Electromyographic signal gesture recognition method based on hidden markov model
An electromyographic signal and hidden Markov technology, which is applied in the field of hidden Markov model recognition of gestures corresponding to electromyographic signals
- Summary
- Abstract
- Description
- Claims
- Application Information
AI Technical Summary
Problems solved by technology
Method used
Image
Examples
Embodiment Construction
[0065] The present invention will be described in further detail below in conjunction with the accompanying drawings and specific embodiments.
[0066] Such as figure 1 Shown, the present invention a kind of method based on Hidden Markov Model judgment myoelectric signal gesture action, concrete implementation steps are as follows:
[0067] Step (1) We use the open data set NinaPro to test the method. The NinaPro data set has rectified and band-pass filtered the signal, and selected 5 wrist movements, 8 sign language gestures and 12 gestures from the gesture set, such as figure 2 shown.
[0068] Step (2) Perform mean value smoothing filtering on the EMG data with a filter window length of 50 ms, and normalize the data. Use the maximum value in each channel of all gesture data of a subject for normalization, the formula is
[0069] X a b s o l u ...
PUM
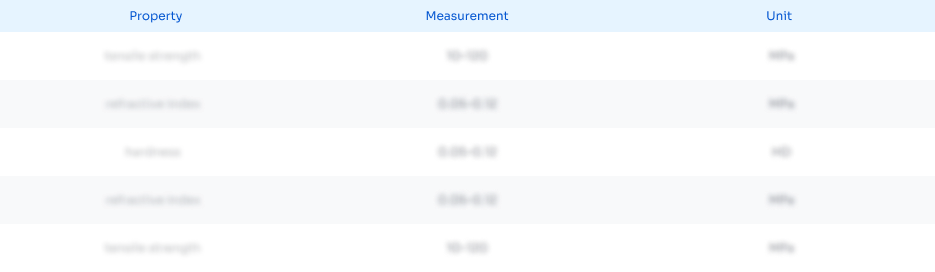
Abstract
Description
Claims
Application Information
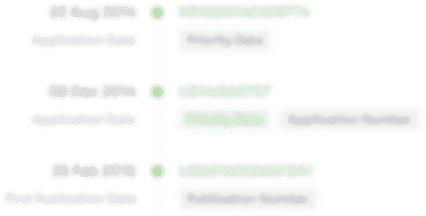
- R&D Engineer
- R&D Manager
- IP Professional
- Industry Leading Data Capabilities
- Powerful AI technology
- Patent DNA Extraction
Browse by: Latest US Patents, China's latest patents, Technical Efficacy Thesaurus, Application Domain, Technology Topic, Popular Technical Reports.
© 2024 PatSnap. All rights reserved.Legal|Privacy policy|Modern Slavery Act Transparency Statement|Sitemap|About US| Contact US: help@patsnap.com