High-accuracy human body multi-position identification method based on convolutional neural network
A convolutional neural network and recognition method technology, applied in the field of multi-part recognition of human body based on convolutional neural network, to achieve the effects of accurate positioning, improved recognition accuracy, and improved accuracy
- Summary
- Abstract
- Description
- Claims
- Application Information
AI Technical Summary
Problems solved by technology
Method used
Image
Examples
Embodiment Construction
[0021] In order to make the purpose, technical solution and advantages of the present invention clearer, the present invention will be further described in detail below in conjunction with the accompanying drawings and formulas.
[0022] figure 2 It is a structural diagram of the convolutional neural network in the overall human body multi-part recognition model of the present invention. It adopts the deep convolutional neural network structure of Krizhevsky et al. The input is a 224*224 RGB image, and then passes through five layers of convolutional layers , three-layer pooling layer and three-layer fully connected layer finally get the classification result of the candidate area. Where the activation function is determined by the previous f(x)=tanh(x) or f(x)=(1+e -x ) -1 It becomes f(x)=max(0,x), which greatly improves the calculation speed of the convolutional neural network. Local response normalization uses b x ...
PUM
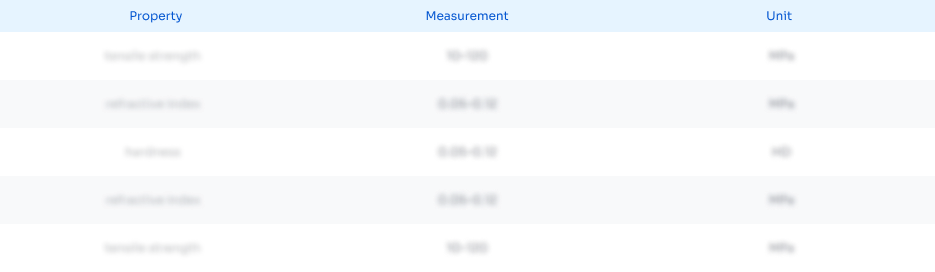
Abstract
Description
Claims
Application Information
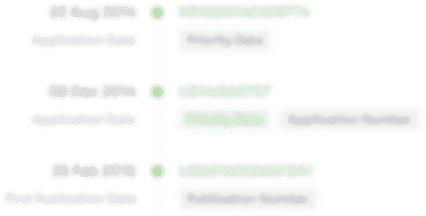
- R&D
- Intellectual Property
- Life Sciences
- Materials
- Tech Scout
- Unparalleled Data Quality
- Higher Quality Content
- 60% Fewer Hallucinations
Browse by: Latest US Patents, China's latest patents, Technical Efficacy Thesaurus, Application Domain, Technology Topic, Popular Technical Reports.
© 2025 PatSnap. All rights reserved.Legal|Privacy policy|Modern Slavery Act Transparency Statement|Sitemap|About US| Contact US: help@patsnap.com