Depth image human body joint positioning method based on convolution nerve network
A technology of convolutional neural network and depth image, which is applied in the field of human joint positioning based on deep image of convolutional neural network, can solve the problem of inability to achieve good results in positioning human joints, difficult integration of bone joint positioning and tracking, and large mechanical noise. question
- Summary
- Abstract
- Description
- Claims
- Application Information
AI Technical Summary
Problems solved by technology
Method used
Image
Examples
Embodiment
[0021] Such as figure 1 As shown, the present invention is based on the deep image human joint positioning method of the convolutional neural network, including the training process and the identification process;
[0022] The steps of the training process are as follows:
[0023] 1) Input training samples;
[0024] 2) Initialize a deep convolutional neural network and its parameters, the parameters including the weight and bias of each layer of edges;
[0025] 3) Using the forward algorithm and the backward algorithm, using the training samples to learn the parameters of the constructed convolutional neural network;
[0026] The steps in the identification process are as follows:
[0027] 4) Input test samples;
[0028] 5) Use the trained convolutional neural network to regress the positions of the human body joints on the input test samples.
[0029] Below in conjunction with concrete technical scheme, technical scheme of the present invention is further elaborated:
...
PUM
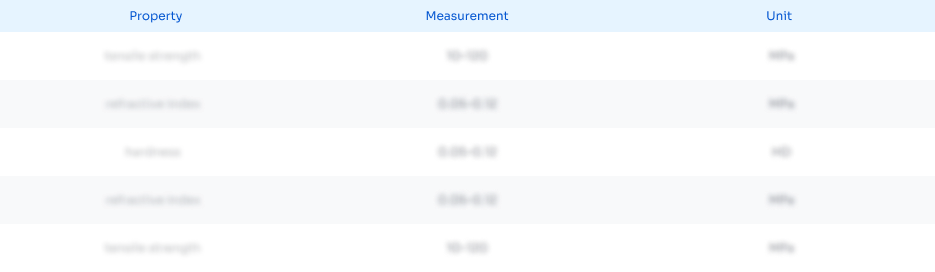
Abstract
Description
Claims
Application Information
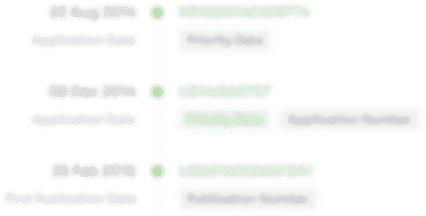
- R&D
- Intellectual Property
- Life Sciences
- Materials
- Tech Scout
- Unparalleled Data Quality
- Higher Quality Content
- 60% Fewer Hallucinations
Browse by: Latest US Patents, China's latest patents, Technical Efficacy Thesaurus, Application Domain, Technology Topic, Popular Technical Reports.
© 2025 PatSnap. All rights reserved.Legal|Privacy policy|Modern Slavery Act Transparency Statement|Sitemap|About US| Contact US: help@patsnap.com