Method for accelerating GPU directed at deep learning super-resolution technology
A technology of super-resolution and deep learning, applied in the field of image super-resolution, can solve problems such as huge computing overhead and cannot meet the needs of practical applications, and achieve the effect of accelerating and optimizing computing speed
- Summary
- Abstract
- Description
- Claims
- Application Information
AI Technical Summary
Problems solved by technology
Method used
Image
Examples
Embodiment Construction
[0023] The present invention will be described in detail below in conjunction with specific embodiments. The following examples will help those skilled in the art to further understand the present invention, but do not limit the present invention in any form. It should be noted that those skilled in the art can make several modifications and improvements without departing from the concept of the present invention. These all belong to the protection scope of the present invention.
[0024] Such as figure 1 Shown, the flowchart of SRCNN. As a preferred embodiment of the present invention, the super-resolution GPU acceleration technology of the present invention is aimed at SRCNN, and its flow chart is as follows figure 1 As shown, it contains bicubic preprocessing (not marked), three convolutional layers and two RELU layers (following the first convolution and the second convolution respectively). The sizes of the three convolutional layers are (according to output channel *...
PUM
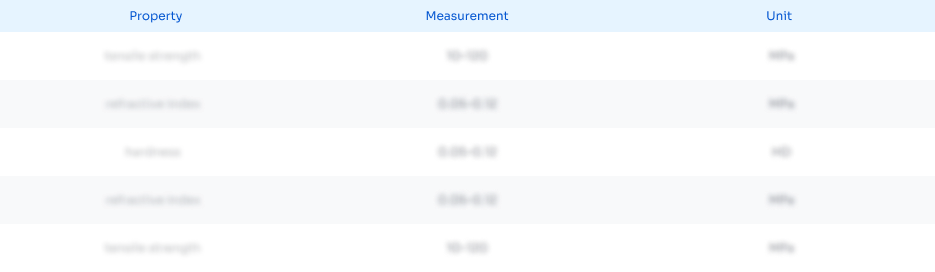
Abstract
Description
Claims
Application Information
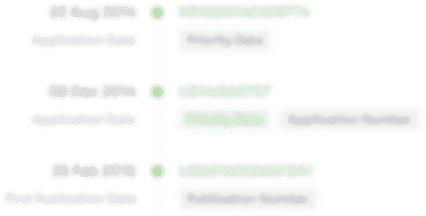
- R&D Engineer
- R&D Manager
- IP Professional
- Industry Leading Data Capabilities
- Powerful AI technology
- Patent DNA Extraction
Browse by: Latest US Patents, China's latest patents, Technical Efficacy Thesaurus, Application Domain, Technology Topic, Popular Technical Reports.
© 2024 PatSnap. All rights reserved.Legal|Privacy policy|Modern Slavery Act Transparency Statement|Sitemap|About US| Contact US: help@patsnap.com