Deep learning and MRI image-based ventricular function index prediction method
A technology of deep learning and prediction methods, which is applied to computer systems based on knowledge patterns, machine learning, character and pattern recognition, etc. It can solve problems such as high labor and time consumption, and human differences.
- Summary
- Abstract
- Description
- Claims
- Application Information
AI Technical Summary
Problems solved by technology
Method used
Image
Examples
specific Embodiment approach 1
[0020] The ventricular function index prediction method based on deep learning and MRI images in this embodiment, combined with figure 1 As shown, the method is realized through the following steps:
[0021] Step 1. Obtain cardiac MRI images in a clinical manner;
[0022] Step 2, manually outline the ventricle and calculate the related ventricular function index;
[0023] Step 3, preprocessing the cardiac MRI image;
[0024] Step 4, using a deep learning method to perform feature representation on the cardiac MRI data;
[0025] Step 5, using machine learning methods to train the ventricular function index prediction model;
[0026] Step 6: Use the trained model to predict the ventricular function index on the cardiac magnetic resonance image obtained in step 1; wherein, MRI refers to nuclear magnetic resonance imaging, and is referred to as magnetic resonance imaging for short.
specific Embodiment approach 2
[0027] The difference from Embodiment 1 is that in the method for predicting ventricular function indicators based on deep learning and MRI images in this embodiment, the cardiac magnetic resonance image in step 1 is a cardiac MRI image acquired by MRI equipment, or two phases and multiple directions Among them, the two phases are the end-systole and the end-diastole, and the slice data in multiple directions are the short-axis tangential image of the base part of the cardiac MRI, the short-axis tangential image of the middle ventricle, and the short-axis tangential image of the upper ventricle , long-axis two-chamber images and long-axis four-chamber images.
specific Embodiment approach 3
[0028] The difference from Embodiment 1 or Embodiment 2 is that in the method for predicting ventricular function indexes based on deep learning and MRI images in this embodiment, the process of manually drawing the outline of the ventricle and calculating the relevant ventricular function indexes described in step 2 is as follows. The ventricular part of the acquired cardiac MRI image is manually segmented, and the ventricular function index is calculated according to the clinical general gold standard; wherein, the ventricular function index specifically includes: the volume, mass, end-systolic blood volume, and diastole of the left and right ventricles. End blood volume and ejection fraction; where mass is calculated from volume and ejection fraction is calculated from end systolic blood volume and end diastolic blood volume.
[0029] Among them, when manually segmenting the ventricle of the cardiac MRI image obtained in step 1, clinicians use general standards, and generall...
PUM
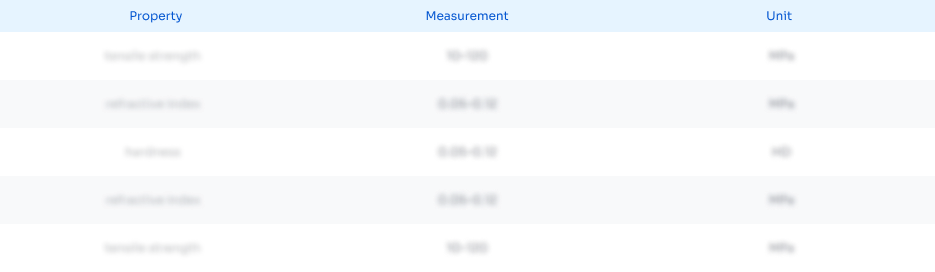
Abstract
Description
Claims
Application Information
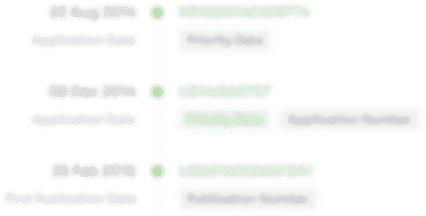
- R&D Engineer
- R&D Manager
- IP Professional
- Industry Leading Data Capabilities
- Powerful AI technology
- Patent DNA Extraction
Browse by: Latest US Patents, China's latest patents, Technical Efficacy Thesaurus, Application Domain, Technology Topic.
© 2024 PatSnap. All rights reserved.Legal|Privacy policy|Modern Slavery Act Transparency Statement|Sitemap