Image noise reduction method utilizing depth full-convolution coding-decoding network
A technology of image noise reduction and convolutional coding, applied in the field of image noise reduction, to achieve the effect of benefiting the network level, optimizing noise reduction performance, and eliminating noise
- Summary
- Abstract
- Description
- Claims
- Application Information
AI Technical Summary
Problems solved by technology
Method used
Image
Examples
Embodiment
[0034] An image denoising method using a deep fully convolutional encoder-decoder network. The input of the model is a noisy image and the output is a clean image after denoising. The model has a very deep encoder-decoder framework. Different from traditional approaches that use prior knowledge of images, this framework directly learns from the training dataset an end-to-end fully convolutional mapping that maps input noisy images to clean output images. The network model consists of multiple layers of convolution and deconvolution operations. Since the deeper the network training is more difficult, this method uses the inter-layer skip connection structure to connect the convolutional layer and the symmetrical deconvolutional layer, so that the training converges faster and a higher quality local optimum can be obtained untie.
[0035] Model framework such as figure 1 As shown, it mainly includes a series of convolutional layers and a symmetrical deconvolutional layer. Th...
PUM
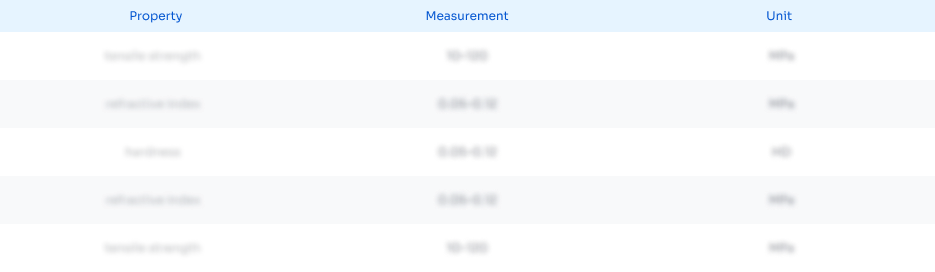
Abstract
Description
Claims
Application Information
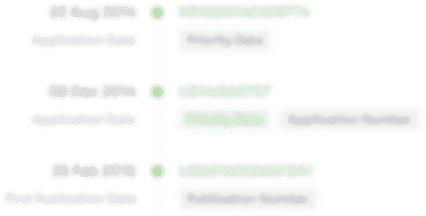
- R&D Engineer
- R&D Manager
- IP Professional
- Industry Leading Data Capabilities
- Powerful AI technology
- Patent DNA Extraction
Browse by: Latest US Patents, China's latest patents, Technical Efficacy Thesaurus, Application Domain, Technology Topic.
© 2024 PatSnap. All rights reserved.Legal|Privacy policy|Modern Slavery Act Transparency Statement|Sitemap