Nonlinear manifold learning dimension reduction method based on adaptive density clustering
A density clustering algorithm and density clustering technology, applied in the fields of instruments, character and pattern recognition, computer parts, etc., can solve the problems of poor reliability, low accuracy, distortion, etc., to overcome the inability to automatically determine and correct High rate and good reliability
- Summary
- Abstract
- Description
- Claims
- Application Information
AI Technical Summary
Problems solved by technology
Method used
Image
Examples
Embodiment Construction
[0036] The present invention will be further described below in conjunction with the accompanying drawings.
[0037] refer to Figure 1 to Figure 5 , a nonlinear popular learning dimensionality reduction method based on adaptive density clustering, has high-dimensional samples M, and each sample of M is a point x, which is usually an object of mathematical representation. Sampling from the embedded low-dimensional fashion (n<
[0038] refer to figure 1 As shown, the nonlinear popular learning dimensionality reduction method based on parallel mapping, the dimensionality reduction method includes the following steps:
[0039] 1) Use the clustering method to form a linear model plane, the process is as follows:
[0040] 1.1 Use the adaptive density clustering algorithm to cluster the data objects in the popular M, automatically dete...
PUM
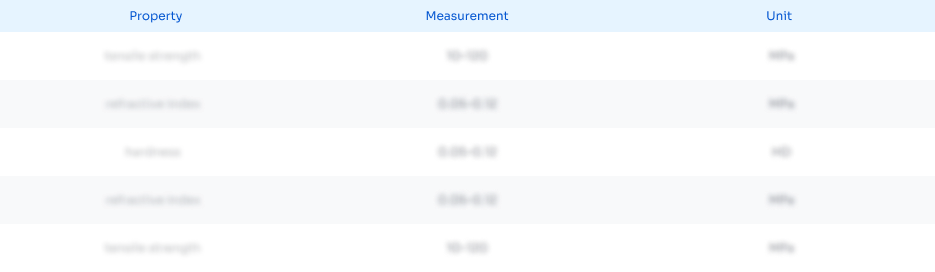
Abstract
Description
Claims
Application Information
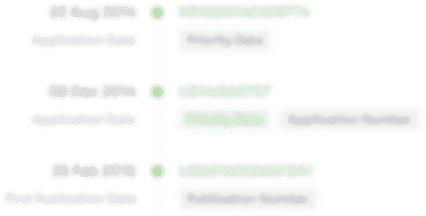
- R&D Engineer
- R&D Manager
- IP Professional
- Industry Leading Data Capabilities
- Powerful AI technology
- Patent DNA Extraction
Browse by: Latest US Patents, China's latest patents, Technical Efficacy Thesaurus, Application Domain, Technology Topic.
© 2024 PatSnap. All rights reserved.Legal|Privacy policy|Modern Slavery Act Transparency Statement|Sitemap